Generative AI: A Journey Towards Sustainable and Human-Centric Productivity
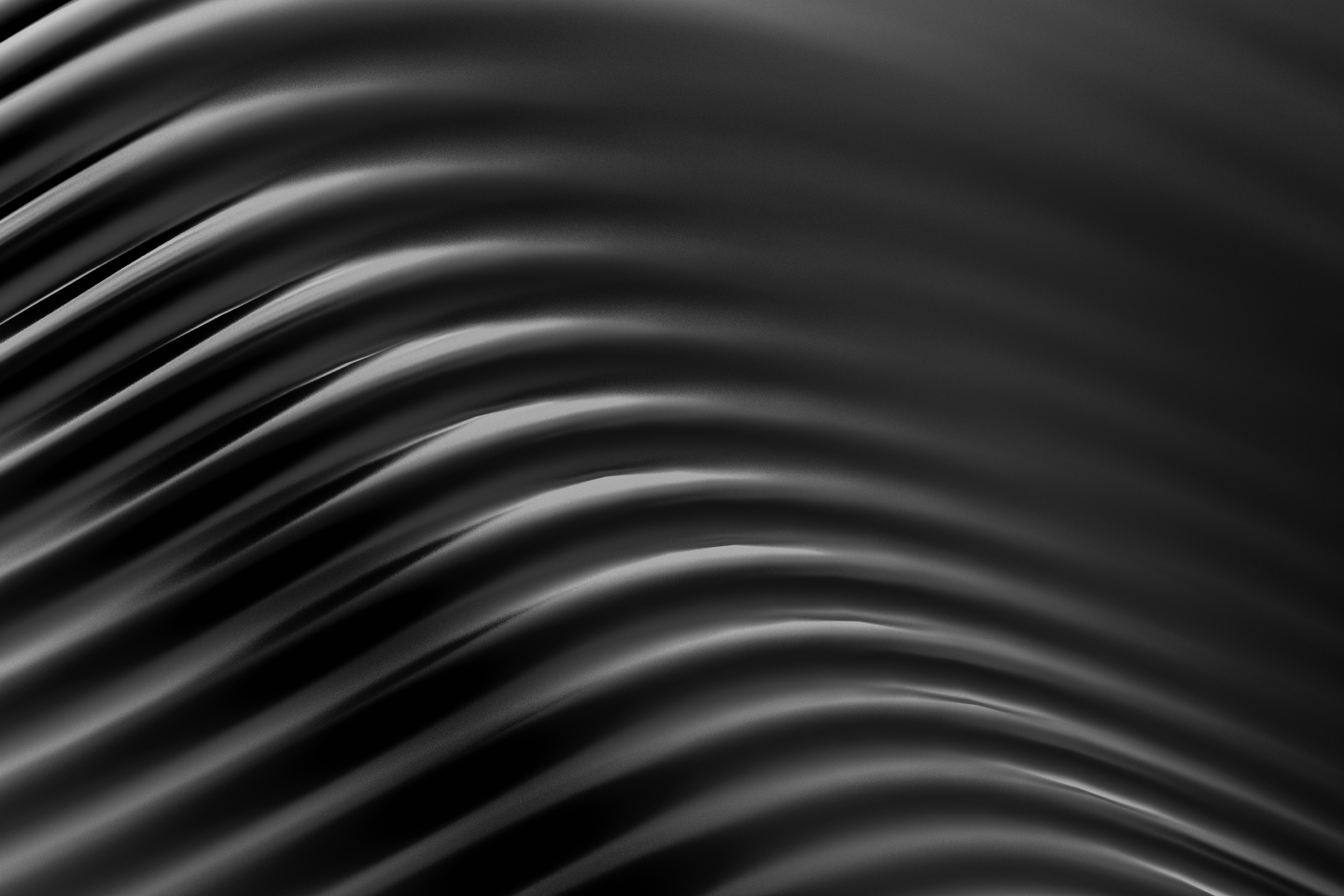
Generative artificial intelligence (AI) has been quite a buzz in the last 12-18 months, and it quite possibly designates the pinnacle of the impact of AI, till date, for humans. There is no doubt that AI has been the most profound disruption in the ever-evolving human pursuit for excellence. It is also true that the recently observed speed of innovation has been outpacing the speed of adoption and stabilization, hence, creating tension and subsequently, a lot of myths and mists which come along with it. It is indeed a time when we, the deep technologists and tech/change leaders come forward to demystify and leverage AI for the wholesome benefit that it brings with it – be it personal productivity, be it enterprise efficiency, and so on.
AI (whether it’s generative, discriminative or others), is about productivity, democratisation, scale, doing more with less and ultimately, giving time back for the greater good. In this article, we will look at what productivity means, what are the necessary guardrails for safe adoption and adaptation and how it is done or should be done given the fact that AI continues to be the second-biggest sustainability/carbon footprint concern after land and air transportation.
Personal Productivity
In the personal productivity space, the use cases have ranged from quick advice through digital assistants, for e.g., Siri, Cortana, Alexa, and Google Assistant for discrete and qualitative enquiries ranging from weather, best restaurants, navigation, and optimised transportation schedules to journey planning, personalised recommendations, and so on. With current advances (a.k.a. generative AI), this has graduated to essay writing, articles, photo or video creation, tour planning, presentation. In a way, this sounds phenomenal right at the onset and overwhelming too as you start analysing the personas who are benefiting(?) from this and hence living the lifecycle emerging from it. As a segue, the following questions become imperative for us to answer and address:
- What will school curriculums look like for our young children of the future, if AI is writing essays or drawing pictures on demand? Who is the real teacher and what will schools be expected to teach?
- How do you define and measure originality of content creation? Recently, the US recording academy has officially banned AI music from being nominated or awarded and is only accepting human compositions.
- Do we differentiate between human-generated content and AI-generated ones, be it text, video or pictures? How do they co-exist, if at all? (E.g., watermarks for AI-generated content?)
- Who owns the authenticity of the generated content or disseminated knowledge? What will the copyrights and trademarks of today change into tomorrow? Or will they even exist at all?
The list goes on, but the fundamental questions are: where do we need AI, why do we need it and what are the benefits it brings? Along with these, there are concerns about malicious, biased, incorrect, or potentially harmful advice given that personal productivity assistants work using public data which lack ownership / accountability and could be outdated or biased. Let’s talk more about that later in the blog.
Enterprise Efficiency
When it comes to enterprises, AI has been seemingly struggling to gain scale and cross the ROI checkpoints, for obvious reasons. Any enterprise or city (mother of enterprises) is a closed ecosystem of multiple complex functions or value streams, and each value stream is executed by a combination of humans, assets and systems working together. For a product, this includes research and design, prototyping, manufacturing, quality control, marketing and sales, servicing, supply chain and so on. Each industry defines these value streams in terms of its products and services and hence domain vocabulary, data ubiquity, product knowledge and market needs become complex triangulation problems that need solving. While there have been pockets of success where AI has transformed product lifecycles into touchless experiences, the following questions still loom large when it comes to AI adaptability in enterprises:
- Which personas and functions in the product lifecycle should be armed with AI-driven productivity? E.g., designers getting 70% ready AI driven engineering drawings. Is it more about product quality or cost reduction?
- Have we eliminated data silos across systems and functions, and do we have quality datasets which will help train AI models? How do we ensure quality data generation? After all, AI is only as good as the data it’s trained on.
- Who is responsible for AI-generated design gaps and false positives in recommendations, especially in safety and mission critical products like aircraft, medical and diagnostic equipment, automobiles, industrial machinery, oil drilling infrastructure, energy grids, communication switches and so on?
- Who owns the IP rights when we generate code thru AI for products and platforms and how do the ownership and indemnities transfer to the actual ISV or IISV? While Engineering foundations are an easy bet to start with, how do we take on non-Functional requirements from an AI generated code and most importantly, create a data set to train for its’ generation?
- How do enabling functions like HR, payroll, leave management, finance/ accounting, executive assistants, and contact centre lend themselves to being totally AI-driven given the fact that they are largely process-oriented, or do we need a human assist for emotion-sensitive rituals like onboarding, separation, promotion, executive decisions? Should we look at an AI driven executive board and directors like Deep Knowledge Ventures in Hong Kong?
The list is much bigger here as every industry is unique in terms how they orchestrate the various functions but the key question hovers around – what are we looking to achieve? Which personas need that productivity boost so that the products/services become futureproof? Which are the personas adding value/innovation as opposed to running repetitive tasks? Or are we looking at pure cost reduction? The domain overlay is a critical necessity for enterprises to succeed with AI, with the core purpose helping to complete the picture.
Exploring Current Practices and Future Prospects
While the why and what of leveraging AI-driven productivity is clearly a matter of choices or guidelines that an individual or an enterprise/it’s persona will make/follow, the ‘how’ part equally influences the final decision and hence the success. In the last few years, a slew of pre-trained transformer engine-based large language models, popularly known as LLMs, have been successful in creating human-like conversations and knowledge dissemination. We have also recently seen that it isn’t only LLMs with gigantic numbers of parameters (176B and counting) that enables rich knowledge exchange; small language models (SLMs) with a comparatively puny 1.3B parameters that were trained on meticulously curated datasets give increased accuracy. Like humans and their training, it’s the quality of the data and its relevance (not necessarily the volume of data) which seems to be defining success. In fact, as we discussed, for enterprises the domain knowledge overlay becomes supercritical and hence a marriage between language models and knowledge (read domain) graphs leading to domain models – aka DMs - whether SDMs or LDMs are potentially achieving some early wins. In any case, for any model (language or domain), the underlying data along with its guard-rails is key. Hence, the following key factors will drive the true efficiency and very importantly its human centricity:
- Data Privacy and Copyright – This is a very sensitive topic as today’s data privacy laws are largely defined by geographical boundaries. Having uniform data privacy laws has been the need of the hour for successful knowledge dissemination. An unvetted training dataset can leak private info and lead to plagiarism.
- Ethics and Bias – Neutralising data bias is extremely critical so that models do not further propagate it. This requires bias checks of the training datasets for imbalances leading to negative consequences. In this regard, the consistency of the model also plays a very important role.
- Responsibility, Explainability, and Ownership – This is another topic where AI-based engines need certification and can potentially be treated at par with human-generated content. Use cases in mission critical and safety critical systems (healthcare, automobiles, aircraft) will unlikely see the light of day unless we establish standards of ownership for AI-generated knowledge.
- Malicious Content and Prompt Injection – Carefully administered prompt engineering can break models, making them prone to generating malicious content like phishing emails and malware. While strong content filters are in vogue, they are yet not fool proof either.
- Is the model architecture green? Are the algorithms green?
- Do we have AI-assisted carbon capture tools like CodeCarbon ++, ML CO2 Impact, which nudges us towards carbon-neutral code generation?
- What does it take to trade-off between 100Billion+ to 100Trillion+ parameters and create textbook-level data sets? How much data we need to store? What is an energy efficient storage?
- What energy sources do we use for training? Renewables or nuclear? Is the time important when the models are trained in terms of carbon intensity?
Harnessing the Power of AI for a Sustainable Future
Today we are fortunate to be at the crossroads of defining a moment of truth for human evolution. It’s very clear that right decisions and choices will pave the way to true human centric success, as it has always been for any technology driven disruptions. Questions are being asked – for humans, will this usher in an age of obsolescence or greater good? As I mentioned earlier, AI’s impact is profound, and it’s in our best interest to embrace it and optimise its potential in a meaningful way with humans and mother Earth at the centre. The path to the greater good is always through obsolescence. Only when we transform our current roles, make them meaningless, we create new avatars of us which is far more evolved, more self-aware – AI is seemingly helping us in that direction, in the process giving us back valuable time to improve ourselves, the society at large and the planet overall.
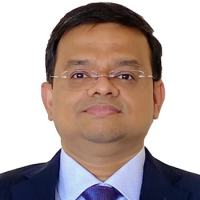
Dhiman Basu Ray is the Chief Technology Officer at Tech Mahindra Engineering Services. Dhiman has more than two decades of business, technology, and engineering transformation experience across verticals with strong foundation in digital technologies. He is an avid thought leader and has been featured in multiple publications and has delivered speaking sessions in forums like CIO Review, Cloud Connect, Data Quest, NASSCOM – to name a few.