Barriers Towards Enterprise AI Adoption - Data Privacy
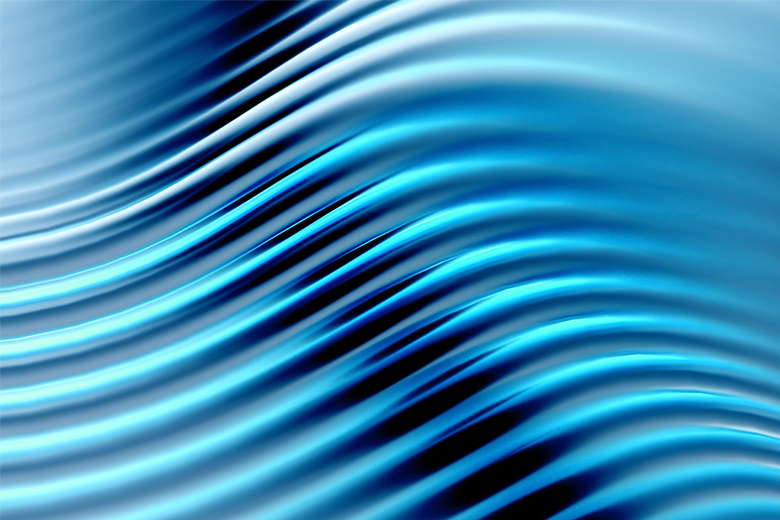
Point of View Series - Part 2
In our inaugural post, we delved into the critical importance of selecting the right business use cases as the cornerstone of any successful AI journey within enterprises. Today, we shift our focus to another formidable barrier standing in the way of widespread AI implementations which is: Data Privacy.
As organizations strive to harness the transformative power of artificial intelligence, they are met with a myriad of challenges, key among them being the protection of sensitive data. In an era where data breaches and privacy concerns dominate headlines, enterprises must navigate a complex maze of regulations, ethical considerations, and consumer expectations to safeguard the privacy of their data assets while leveraging AI technologies to drive innovation and growth.
Barrier # 2 - Data Privacy
Data privacy awareness drives responsible management and protection of sensitive information throughout its lifecycle – from data creation, collection, storage, processing, sharing, to disposal. It ensures that sensitive data, including personal and business data, is protected from loss, misuse, unauthorized access, or improper disclosure.
It involves legal compliance, ethical considerations, security measures, and transparency to build trust with customers, employees, and stakeholders.
Now, why is this important when organizations are exploring AI adoption?
AI systems are trained with large amounts of contextual data, which could include customer personal information or financial data that may have been potentially breached knowingly or unknowingly, compromised, or collected from users without their knowledge or consent. Data sourced through such channels, even unconsciously, could be associated with lawsuits involving identity theft or fraud that might drag in enterprises, due to association, if they are using the same datasets - raising concerns about how they are being used and shared with third parties.
Additionally, the models, which learn from all kinds of data (sensitive and otherwise), will respond based on the queries they are subjected to post-training. They cannot differentiate and hence cannot vary their response based on where the queries come from, whether from good actors or bad actors.
Protecting these models, for both the above reasons (wrong inputs during training and wrong outputs during querying), becomes as important as protecting the data to which they are exposed. Model management, model governance, and lifecycle management of models hence play a vital role in protecting such sensitive data and information exposure.
It is possible for enterprises to take some concrete steps to mitigate this concern.
- First, they must audit the externally sourced training dataset (and the source thereof) being used to train the AI algorithms they are thinking of using.
- Second, they need to implement strong data security measures (including technologies like encryption for data at rest and in transit and programs like Data Protection Impact Assessment – DPIA, and Privacy Impact Assessment – PIA) to protect from unauthorized access, theft, or loss while also having strong policies governing data retention and deletion.
- Third, they need to transparently disclose how they are planning to use the data and/or share it with third parties, implementing strict data access controls to allow such usage (view/modify/delete) post-authorization while also implementing data traceability and audit mechanisms to ensure that third parties are using the shared data exactly as allowed.
- Finally, they must give users control over their data and allow them to choose how they are used, including taking their consent each time they are used. This will go a long way in building trust.
Some such actions are increasingly being addressed either through compliance efforts directed at regulations (like GDPR in the EU, CCPA and HIPAA in the US, LGPD in Brazil, POPIA in South Africa) or awareness and acceptance of ethical considerations. Newer approaches like Privacy by Design, where data privacy safeguards are built into every stage of product or service design and development lifecycles, also help here.
Other technologies like federated learning, homomorphic encryptions, and differential privacy, which allow AI systems to learn from data without accessing sensitive information, can strengthen an enterprise’s posture against potential data privacy issues.
Even after implementing all the above, it would still be important to invest in employee training and awareness as they play a crucial role in maintaining data privacy. However, if and when such privacy breaches happen, there should be a well-defined incident response and reporting structure to limit and minimize impact and damages as well as recover from these incidents.
Conclusion
To sum up, this blog focuses on two key elements of data privacy in the context of enterprise AI implementations – (1) Why is it important and (2) What can enterprises do to address the risks associated with it. In today's digital landscape, enterprises embarking on AI journeys must prioritize data privacy to ensure the responsible management of sensitive information. This encompasses the entire data lifecycle, from creation to disposal, and requires adherence to legal standards, ethical practices, robust security protocols, and transparency. AI systems, reliant on vast contextual datasets, may inadvertently utilize compromised or unauthorized data, raising legal and ethical concerns.
Hence, safeguarding AI models against misuse is as crucial as protecting the data itself. Enterprises can mitigate these risks by rigorously auditing training datasets, implementing strong security measures like encryption, conducting data protection impact assessments, and maintaining strict data governance policies. Transparent disclosure of data usage, stringent access controls, and enabling user consent empower trust building. Compliance with global regulations, ethical considerations, and the adoption of privacy by design principles further reinforce data privacy. Technologies like federated learning and homomorphic encryption enhance the privacy of AI systems. Despite these precautions, continuous employee training and robust incident response plans are essential to minimize the impact of any potential data breaches.
In the next post of our blog series, you will see us addressing the topic of ‘Data Quality and Quantity’. In enterprise AI journeys, data quality ensures the accuracy, relevance, and reliability of AI-driven decisions, while data quantity enables the development of robust, generalizable models. Together, they form the backbone of successful AI initiatives, facilitating sophisticated insights, driving continuous improvement, and enabling competitive advantage in the marketplace.
If you missed the first post of the series, you could find it here
KNOW MORE
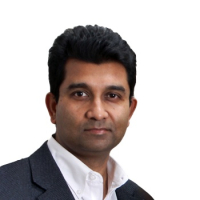
Partha currently manages an industry business group of strategic lighthouse customer relationships within the TME business unit at Tech Mahindra. He brings over two and a half decades of experience in discrete manufacturing and technology consulting services covering North America, Europe, and Asia Pacific markets across automotive, consumer electronics, semiconductor, networking, ISVs, gaming and financial services domains.More
Partha currently manages an industry business group of strategic lighthouse customer relationships within the TME business unit at Tech Mahindra. He brings over two and a half decades of experience in discrete manufacturing and technology consulting services covering North America, Europe, and Asia Pacific markets across automotive, consumer electronics, semiconductor, networking, ISVs, gaming and financial services domains. In his professional career he has helped to design and execute multiple business value impact strategies while managing strategic client relationships and industry vertical focused P&L Management responsibilities.
Less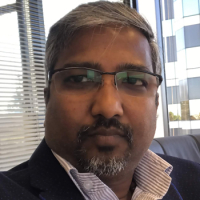
Dr. Pandian Angaiyan heads Tech Mahindra’s technology business as the chief technology officer (CTO) and is based out of San Jose office. He has three decades of experience in incubating and leading computing businesses based on niche technologies, which gives him the right tools to lead disruptive digital transformation initiatives for Tech Mahindra’s customers.More
Dr. Pandian Angaiyan heads Tech Mahindra’s technology business as the chief technology officer (CTO) and is based out of San Jose office. He has three decades of experience in incubating and leading computing businesses based on niche technologies, which gives him the right tools to lead disruptive digital transformation initiatives for Tech Mahindra’s customers. In his previous role, he has lead the cloud Innovation business for a global consulting company where he has played the role of cloud transformation partner for several customers, helping define their cloud strategy, building minimum viable products, and eventually transforming them into full- fledged solutions. Dr. Pandian has two decades of experience in various computing technologies starting from embedded systems all the way to hyper scale architectures.
Dr. Pandian has a Ph.D., in symbolic and numeric computational algorithms for real time applications from the Indian Institute of Science, Bangalore and has a masters of technology in computer engineering from Mysore University, India.
Less