Barriers Towards Enterprise AI Adoption - Data Quantity and Quality
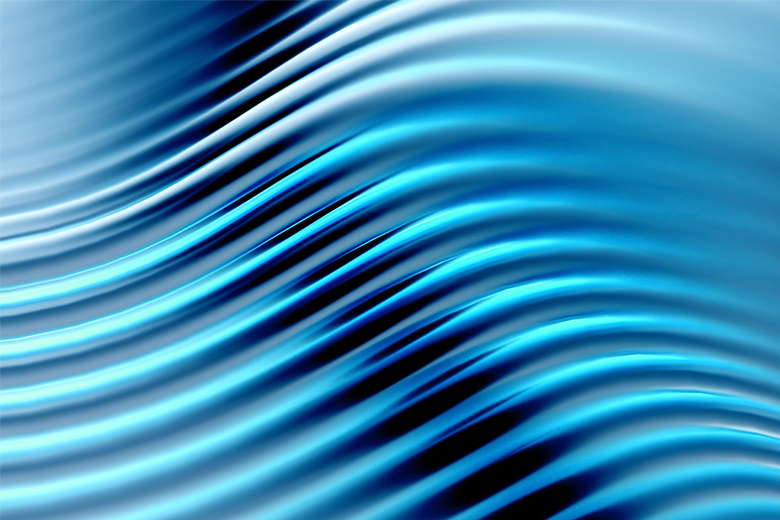
Point of View Series - Part 3
In our earlier two posts, we touched upon the importance of selecting the right business use cases and the demands of data privacy as two potential barriers towards enterprise AI implementations. In this post, we would spend time on the third barrier duo: data quantity and quality.
Data is the fuel which runs the AI engines (models). The right quantity and quality of data is the bedrock upon which AI algorithms and models are built, trained, and deployed. The challenges of managing these two critical data attributes are multifaceted, spanning from broad, systemic issues to more granular, technical obstacles. Let’s start understanding what these challenges are and what can enterprises do to overcome them.
Barrier # 3 - Data Quantity and Quality
The right quantity and quality of data needs and their associated challenges are both – independent as well as interdependent. Hence to understand them we need to first look at their nuances separately before looking at how they interact with each other.
Let’s start with data quantity first
The top three macro challenges which influence this attribute are scalability of existing data infrastructure, need for data diversity and the cost associated with collecting the right volumes of data. As AI models become more complex, the volume of data required for training and inference grows exponentially, necessitating a robust infrastructure capable of storing, processing, and managing vast number of datasets. These datasets must also be diverse enough to appropriately encompass a wide range of scenarios, variables, and conditions. Finally, the costs associated with acquiring, storing, and processing such large volumes of diverse data can be prohibitive and could potentially hit enterprise’s budgetary constraints.
Assuming that an enterprise sets up the right infrastructure, maps the best possible diverse datasets and secures the budget for implementation , they would still need to understand and need to have a strategy to address the next set of micro challenges associated with data collection and acquisition, data labeling and annotation, data storage and management, data cleansing and pre-processing, data decay, data privacy and compliance, data integration across multiple sources and data redundancy amongst other operating factors.
Now let’s focus on the data quality dimension
At a macro level we need to be aware of volume and variety dynamics, technological heterogeneity and associated regulatory and compliance issues. big data, characterized by its volume, velocity, and variety, complicates the management, processing, and analysis necessary for effective AI implementations. Managing this deluge of structured and unstructured data from disparate sources requires robust systems and processes. Enterprises mostly bring in a legacy of multitude of technologies across departments and functions, leading to a fragmented technological landscape. This heterogeneity complicates the integration, aggregation, and harmonization of data, impeding the creation of a unified data ecosystem.
Finally, enterprises today must navigate an increasingly complex and stringent regulatory landscape that governs data privacy, protection, and usage which adds layers of complexity to data management and quality assurance efforts.
After enterprises figure out a way to manage the above, it then boils down to first developing an appreciation and thereafter setting appropriate data quality assurance processes to collect and manage good quality data. In our experience we see the following 10 micro elements of data getting the focus and care in recent times – diversity, consistency, accuracy, completeness, duplicity, timeliness/recency, relevancy, standardization, integrity, and security.
Quality and quantity have interesting symbiotic relationships too
Good quality data can reduce the need for AI algorithms to have higher data volumes to be effective. Larger data volumes, on the other hand, can allow less than ideal data quality inputs to still work by allowing a wider spectrum of scenarios to be trained and tested. The balance is dependent on the chosen use case and takes multiple iterations to reach the perfect equilibrium. Enterprises embarking on AI journeys must navigate this balance carefully. An overemphasis on quantity, with a disregard for quality, can lead to "garbage in, garbage out" scenarios, where AI models produce unreliable or biased outcomes. Conversely, focusing too narrowly on quality may result in overly constrained datasets that lack the diversity and breadth necessary for effective AI learning.
"The key is to recognize that data quality and quantity are not mutually exclusive but mutually reinforcing."
How Do Organizations Manage These Data Challenges And Strike These Harmonious Relationships?
Enterprises embarking on AI initiatives gain significant value from adopting frameworks like CRISP-DM (Cross-Industry Standard Process for Data Mining), DataOps, TDWI (Transforming Data with Intelligence) data management maturity model, DAMA-DMBOK (International Data Management Body of Knowledge), and FAIR ( findability, accessibility, interoperability, and reusability) data principles, as these frameworks provide structured approaches to enhance both data quality and quantity, which are crucial for successful AI deployments.
CRISP-DM, for example, offers a comprehensive methodology for data mining projects, ensuring that data handling processes are well-defined and repeatable. DataOps emphasizes the importance of communication, collaboration, and automation in data analytics, enabling more efficient and error-free data flows. The TDWI data management maturity model helps organizations assess their data management practices and identify areas for improvement, guiding them toward best practices in data handling. DAMA-DMBOK serves as an extensive guide for data management professionals, covering a broad range of topics necessary for maintaining high data quality and accessibility. FAIR data principles advocate for data to be findable, accessible, interoperable, and reusable, ensuring that data assets are easily shared and leveraged across various applications and initiatives.
"By integrating some of these frameworks into their data management strategies, enterprises can not only standardize and improve their data handling processes but also lay a solid foundation for leveraging AI technologies effectively, leading to more reliable insights, better decision-making, and enhanced competitive advantage.
Other emerging frameworks and methodologies that complement these efforts include MLOps, which focuses on automating and optimizing machine learning lifecycle management, and data mesh, which emphasizes decentralized data ownership and architecture to improve data accessibility and quality at scale.
Conclusion
To sum up, data serves as the critical fuel for AI models, with its quantity and quality forming the foundational elements for building, training, and deploying these algorithms. However, balancing these aspects is complex, with challenges like infrastructure scalability, data diversity, and cost on one hand and volume & variety dynamics, technological heterogeneity and associated regulatory and compliance issues on the other.
Enterprises must navigate multiple macro issues while staying focused on several micro elements. Striking the right balance between data quantity and quality is, hence, pivotal. While high-quality data may reduce volume needs, ample data can compensate for quality deficiencies. To manage these challenges, organizations employ frameworks like CRISP-DM (Cross-Industry Standard Process for Data Mining), DataOps, TDWI (Transforming Data with Intelligence) data management maturity model, DAMA-DMBOK (International Data Management Body of Knowledge), and FAIR ( findability, accessibility, interoperability, and reusability) Data principles to maintain the delicate equilibrium essential for successful AI implementations.
If you missed the first post of the series, you could find it here
KNOW MORE
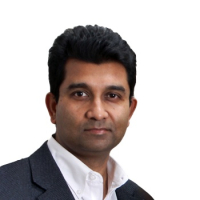
Partha currently manages an industry business group of strategic lighthouse customer relationships within the TME business unit at Tech Mahindra. He brings over two and a half decades of experience in discrete manufacturing and technology consulting services covering North America, Europe, and Asia Pacific markets across automotive, consumer electronics, semiconductor, networking, ISVs, gaming and financial services domains.More
Partha currently manages an industry business group of strategic lighthouse customer relationships within the TME business unit at Tech Mahindra. He brings over two and a half decades of experience in discrete manufacturing and technology consulting services covering North America, Europe, and Asia Pacific markets across automotive, consumer electronics, semiconductor, networking, ISVs, gaming and financial services domains. In his professional career he has helped to design and execute multiple business value impact strategies while managing strategic client relationships and industry vertical focused P&L Management responsibilities.
Less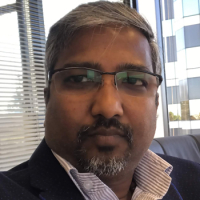
Dr. Pandian Angaiyan heads Tech Mahindra’s technology business as the chief technology officer (CTO) and is based out of San Jose office. He has three decades of experience in incubating and leading computing businesses based on niche technologies, which gives him the right tools to lead disruptive digital transformation initiatives for Tech Mahindra’s customers.More
Dr. Pandian Angaiyan heads Tech Mahindra’s technology business as the chief technology officer (CTO) and is based out of San Jose office. He has three decades of experience in incubating and leading computing businesses based on niche technologies, which gives him the right tools to lead disruptive digital transformation initiatives for Tech Mahindra’s customers. In his previous role, he has lead the cloud Innovation business for a global consulting company where he has played the role of cloud transformation partner for several customers, helping define their cloud strategy, building minimum viable products, and eventually transforming them into full- fledged solutions. Dr. Pandian has two decades of experience in various computing technologies starting from embedded systems all the way to hyper scale architectures.
Dr. Pandian has a Ph.D., in symbolic and numeric computational algorithms for real time applications from the Indian Institute of Science, Bangalore and has a masters of technology in computer engineering from Mysore University, India.
Less