Barriers Towards Enterprise AI Adoption - Right Use Case Finalization
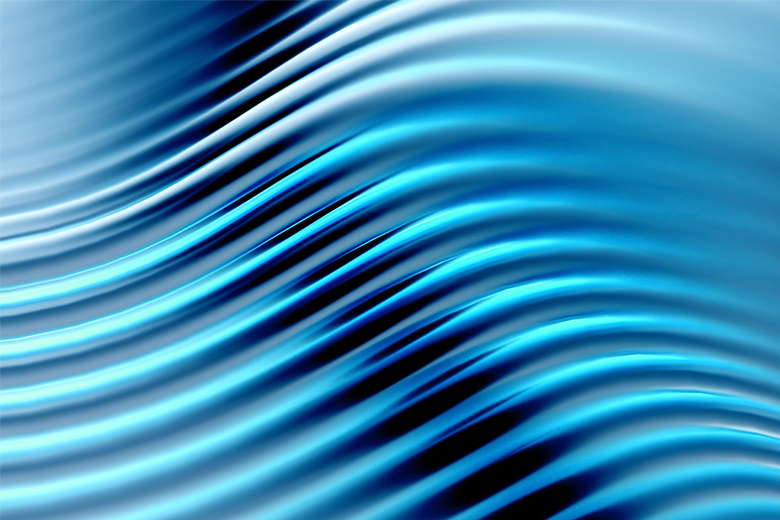
Point of View Series - Part 1
Enterprise AI adoption is an ambitious journey that organizations embark upon to harness the transformative power of artificial intelligence (AI) technologies within their business operations. While the promise of AI is undeniable, the path to successful adoption is riddled with challenges.
Today it is possible to come across a lot of literature on the potential and promise of AI. However, to reach that desired end state, successful implementation and adoption of AI must happen first. That requires addressing and navigating through multiple challenges and barriers, a topic which has not seen too much focus and attention.
Our effort towards writing this blog series is to focus on the less glamorous but more important area of challenges and barriers management in the context of enterprise AI initiatives. As we delve deeper into this series, we will explore a number of significant barriers that hinder these initiatives and talk about how to address / manage them effectively and efficiently thereby improving the chances of making such Enterprise AI journeys successful.
The value of any enterprise technology (and AI is not any different) comes from its appropriate application to help enterprises do business better. Ensuring the right technology and use case marriage is a crucial first step in designing such technology road maps. The first barrier we would hence be talking about, as we kick start this blog series, is the importance of right use case finalization.
Barrier # 1 > Right use case finalization
The key to starting an AI journey is the ability to separate hype from reality. Finalizing the right use cases that are aligned with organizational goals becomes very important and must be backed up with adequate investments and resources.
This requires essentially two things –
1.Understanding of AI capabilities which are mature enough and have seen higher levels of enterprise-wide adoption across industry segments.
2.Finalizing the right use cases which can leverage these capabilities through a process of identification and prioritization.
,The AI capabilities which are reaching some acceptable levels of maturity today can help with tasks like understanding (from image/text/audio/video), extraction, classification / clustering / association, optimization, summarization, estimation, personalized recommendation, and subsequent action automation (like task automation, movement automation, decision automation)
These capabilities are getting tapped into successfully within enterprises to do one or more of the following things today –
- Improve process architecture which drives higher customer satisfaction > By improving existing products / services to serve customers better.
- Improve process efficiency which lowers cost of operations >Automate tasks/processes to cut down time and / or errors.
- Expand revenue sources which increases the ability for higher customer spend capture > Create new products/services/markets to either offer more to existing customers or find new customers and segments.
Identification first and prioritization next, is a multi-parameter led consideration approach. These parameters are indicative, and enterprises can enrich them with more as they find relevant.
It is important to, however, understand the difference between identification and prioritization in this context:
Use case identification (Should we do?) Must consider – strategic relevance (create savings, generate revenues or both), strategic alignment (with enterprise business), industry adoption and success rates, industry direction, ethics and regulatory implications.
Use case prioritization (Can we do?) Must consider – risk vs return, technology and infrastructure availability and access, process readiness, data availability and access (volume, variety, quality), ease of implementation (talent, time, cost) and integrations (with existing systems), internal stakeholder support, scalability potential, impact on employees, and overall returns.
Once you have identified the potential use cases, a quick handy reference to help you prioritize would be as below consisting of a typical 2X2 efforts vs returns (ER) and scalability vs complexity (SC) metrices.
1. Efforts Vs Returns (ER) Matrix
The effort vs returns will help in focusing on the use cases starting with low effort and high returns followed by high efforts and high returns. The below diagram provides few examples of the use cases in each quadrant. When prioritizing AI use cases, organizations typically focus on the ;low effort, high returns’ and ‘high effort, high returns’ quadrants, as these areas are more likely to provide the best balance between impact and investment. However, it's important to consider the specific context, strategic goals, and available resources of the enterprise to make informed decisions about which use cases to pursue.
Low Efforts and High Returns | High Efforts and High Returns |
These are quick wins that can provide significant value to the organization without requiring a substantial investment. Examples:
| While they may be more challenging use cases from implementation perspective, the potential returns justify the investment. Examples:
|
Low Efforts and Low Returns | High Efforts and Low Returns |
While the use cases may be easy to implement, the impact on the organization's bottom line is limited. Examples:
| These use cases should be carefully evaluated, as the investment may not be justified given the expected returns. Examples:
|
2. Scalability Vs Complexity (SC) Matrix
For most organizations, ‘low complexity, high scalability’ and ‘high complexity, high scalability’ use cases are of primary interest, as they offer a balance between technical sophistication and the potential to deliver a broad impact across the organization. The below diagram shares the quadrants and examples for prioritization:
Low Complexity and High Scalability | High Complexity and High Scalability |
AI use cases that are relatively simple to implement and maintain, but they offer high scalability. These use cases can be quickly extended to handle a large volume of data or users and can adapt easily to evolving business needs. Examples:
| These use cases involve advanced AI algorithms and models that can handle substantial data and user loads. While they may be challenging to develop and maintain, their scalability justifies the investment. Examples:
|
Low Complexity and Low Scalability | High Complexity and Low Scalability |
The use cases are relatively simple and don't require advanced AI techniques, but they lack the potential for extensive scalability. These use cases might be suitable for addressing specific, smaller-scale problems. Examples:
| The use cases that are both complex and inherently limited in scalability. These use cases may be resource-intensive to develop and maintain and applicable to a niche problem or a specific, well-defined audience. Examples:
|
Conclusion
This post should have helped you to understand the importance of “Right use case finalization” and provide you with a scientific construct to approach this better.
The next post will focus on the topic of data privacy which will help readers to understand that managing data privacy is crucial in the context of enterprise AI initiatives because it safeguards sensitive information, maintains legal compliance, and fosters trust. Enterprises often handle vast amounts of data, including customer and employee data, and AI relies heavily on this data for decision-making. Mishandling or exposing this data can lead to severe consequences, including legal penalties and damage to reputation. Enterprises have to be aware of what can go wrong and prepare themselves appropriately.
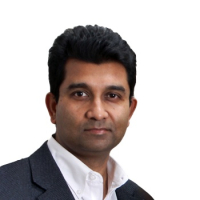
Partha currently manages an industry business group of strategic lighthouse customer relationships within the TME business unit at Tech Mahindra. He brings over two and a half decades of experience in discrete manufacturing and technology consulting services covering North America, Europe, and Asia Pacific markets across automotive, consumer electronics, semiconductor, networking, ISVs, gaming and financial services domains.More
Partha currently manages an industry business group of strategic lighthouse customer relationships within the TME business unit at Tech Mahindra. He brings over two and a half decades of experience in discrete manufacturing and technology consulting services covering North America, Europe, and Asia Pacific markets across automotive, consumer electronics, semiconductor, networking, ISVs, gaming and financial services domains. In his professional career he has helped to design and execute multiple business value impact strategies while managing strategic client relationships and industry vertical focused P&L Management responsibilities.
Less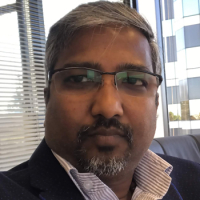
Dr. Pandian Angaiyan heads Tech Mahindra’s technology business as the chief technology officer (CTO) and is based out of San Jose office. He has three decades of experience in incubating and leading computing businesses based on niche technologies, which gives him the right tools to lead disruptive digital transformation initiatives for Tech Mahindra’s customers.More
Dr. Pandian Angaiyan heads Tech Mahindra’s technology business as the chief technology officer (CTO) and is based out of San Jose office. He has three decades of experience in incubating and leading computing businesses based on niche technologies, which gives him the right tools to lead disruptive digital transformation initiatives for Tech Mahindra’s customers. In his previous role, he has lead the cloud Innovation business for a global consulting company where he has played the role of cloud transformation partner for several customers, helping define their cloud strategy, building minimum viable products, and eventually transforming them into full- fledged solutions. Dr. Pandian has two decades of experience in various computing technologies starting from embedded systems all the way to hyper scale architectures.
Dr. Pandian has a Ph.D., in symbolic and numeric computational algorithms for real time applications from the Indian Institute of Science, Bangalore and has a masters of technology in computer engineering from Mysore University, India.
Less