From Vision to Value: The Role of Outcome-Centric Governance in GenAI Adoption
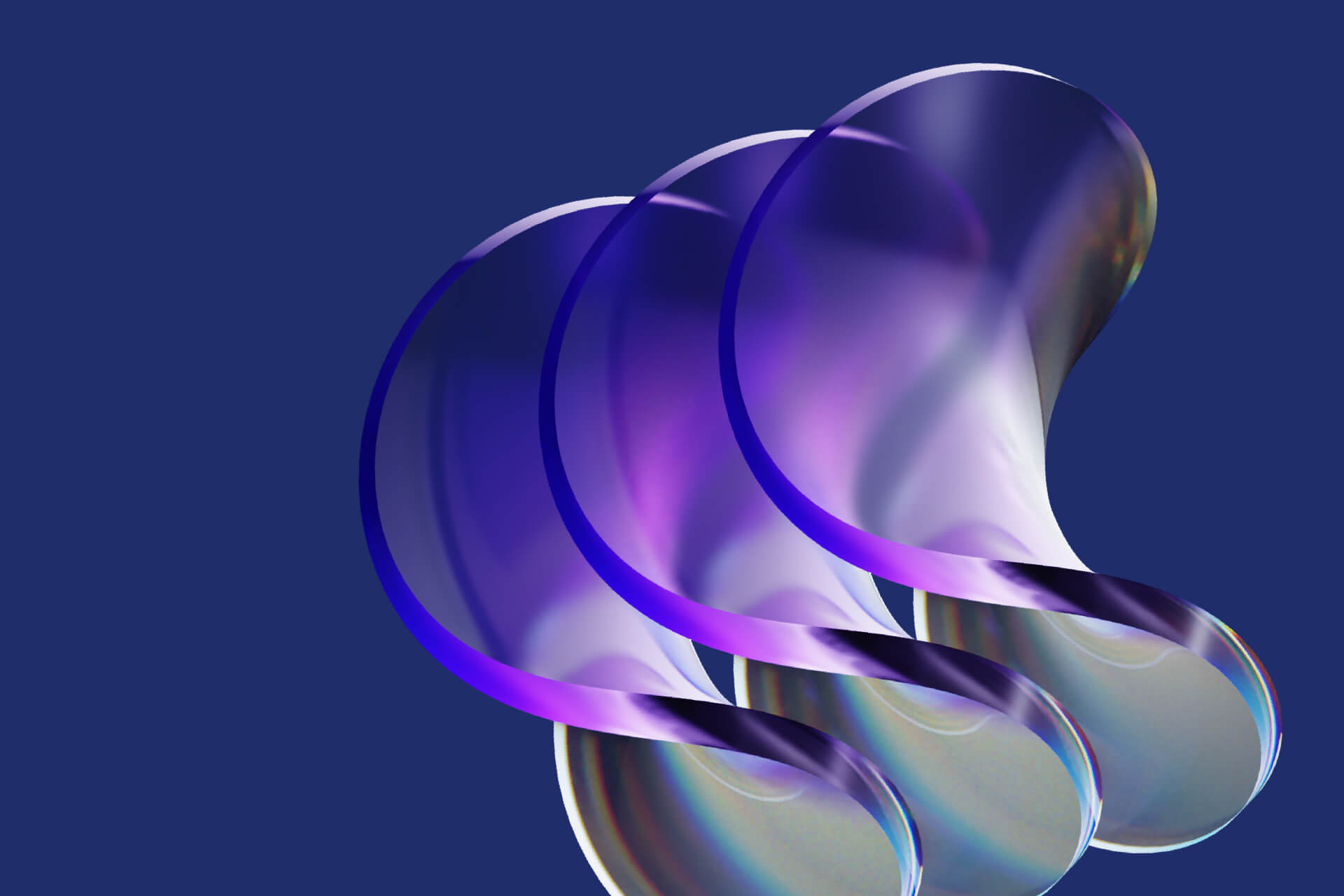
From automating customer service to optimizing supply chains, Generative AI (GenAI) is transforming operations, enhancing efficiency, and driving innovation. However, harnessing its full potential requires more than just implementing cutting-edge technology. It needs strategic and thorough management. This is where outcome-centric governance emerges as a crucial factor in ensuring the success of GenAI initiatives within enterprises.
At Tech Mahindra we are partnering with Microsoft to provide the full potential of GenAI capabilities. This collaboration helps enterprises increase effectiveness and personalization by using GenAI. It also unlocks the full potential of enterprise data, strong governance, and value realization for clients.
We have an integration with Microsoft to enable GenAI-powered Enterprise Knowledge Search, which comes under Tech Mahindra’s TechM amplifAI0->∞ suite of AI offerings and solutions. It helps enterprises increase effectiveness and personalization by using GenAI for unlocking the full potential of enterprise data and a multi-modal, multi-channel search experience. Our GenAI-powered Enterprise Knowledge Search integrates Microsoft Azure OpenAI Service, Azure Cognitive Search, and Azure Language Understanding to help enterprises unleash knowledge accessibility in a unique way, which eventually improves the knowledge quotient within organizations. This brings multiple AI-led capabilities like content summarization, knowledge graph-led knowledge structuring, and a new kind of query interface.
Tech Mahindra helps enterprises accelerate business transformation and increase effectiveness and personalization by using GenAI and Microsoft 365 Copilot responsibly. Our experimentation platform, GenAI Studio, helps customers fast-track exploration of use cases and ensure real value gains. Tech Mahindra helps businesses amplify human ingenuity, creativity, and productivity with a copilot for everyone.
With Tech Mahindra and Microsoft, enterprises can safeguard their business and data with the most trusted AI platform in a responsible manner.
The Imperative of Outcome-Centric Governance
Outcome-centric governance moves away from traditional governance models, which are more about risk management and process adherence over aligning with strategy and achieving clear outcomes. Outcome-centric governance revolves around flexibility, continuous alignment with business objectives, and a focus on delivering specific, quantifiable results. This approach is essential for turning the vast potential of GenAI into real business impact.
The Essence of Outcome-Centric Governance
At its core, outcome-centric governance ensures that every GenAI initiative is precisely designed, executed, and evaluated based on its ability to deliver specific business outcomes. This involves several key elements, including setting clear objectives, defining relevant use cases, establishing a comprehensive governance framework, continuously monitoring performance, engaging stakeholders, and addressing ethical and legal considerations.
Clear Objectives and Use Cases
A fundamental pillar of outcome-centric governance is setting clear objectives and prioritizing use cases. Enterprises must start by carefully defining their goals with their GenAI initiatives, identifying key performance indicators (KPIs), and outlining desired business impacts. These objectives should adhere to the SMART criteria—specific, measurable, achievable, relevant, and time-bound—to ensure clarity and effectiveness. Subsequently, it is important to prioritize use cases that closely align with these strategic goals and promise significant returns on investment (ROI).
Imagine a retail powerhouse aiming to boost customer engagement by 20% annually through GenAI-driven personalized recommendations. This could result in tailored email campaigns and dynamic website content, based on individual customer preferences. By focusing efforts and resources on specific, high-impact initiatives, enterprises can avoid dilution and maximize the effectiveness of GenAI implementations.
Comprehensive Framework
A robust governance framework is important for guiding the deployment and management of GenAI initiatives. This should include flexible yet stringent guidelines outlining the scope, permissible data sources, and acceptable uses of GenAI. While flexibility drives innovation and responsiveness to new insights, preventing misuse and ensuring strict compliance with ethical standards is essential. Moreover, robust access control mechanisms are another critical component of outcome-centric governance. These mechanisms ensure that only authorized personnel can interact with GenAI systems and data, thereby safeguarding against unauthorized access and misuse. This involves a crystal-clear delineation of roles and responsibilities, implementing strict user authentication protocols, and a regular review of access permissions to maintain integrity and compliance standards.
Monitoring and Adaptability
Continuous monitoring and adaptability are the cornerstones of outcome-centric governance. Enterprises must consistently track the performance of GenAI applications against defined objectives and KPIs to assess their efficacy. This involves gathering and analyzing data to determine if AI systems are producing the expected results. If performance deviates from targets, prompt corrective actions should be taken to realign the initiatives with business goals.
Furthermore, the governance framework is not a rigid structure; it's a dynamic system that ensures GenAI initiatives remain relevant and effective. The governance model should have dynamic capabilities, allowing it to evolve with emerging insights, technological advancements, and changing business needs. This adaptability not only facilitates long-term success but also enables enterprises to remain agile and responsive amidst a rapidly changing landscape, instilling confidence in their ability to handle emerging challenges.
Take, for instance, a financial institution leveraging GenAI for fraud detection. In this scenario, it must proactively predict and prepare for the emerging new fraud modalities. Therefore, the governance framework must include provisions for continuous updates to AI models and training data. This ensures perpetual alignment with the evolving threat landscape and sustains effectiveness and relevance.
Stakeholder Engagement
Engaging stakeholders from diverse organizational domains is not just a formality; it's a fundamental pillar of successful GenAI governance. This involves fostering collaboration and soliciting input from representatives across departments, including IT, data science, legal, compliance, and various business units. By incorporating diverse perspectives and expertise, enterprises can ensure GenAI initiatives are fully aligned with strategic objectives and foster a culture of shared ownership and collaboration. This helps each stakeholder feel valued and integral to the success of GenAI initiatives.
Regular reviews with stakeholders help assess progress, address challenges and refine the governance model. These reviews should be structured yet flexible, facilitating open dialogue and collective problem-solving. By involving stakeholders in the governance process, enterprises can enhance transparency, accountability, and buy-in, promoting collaboration and shared responsibility.
Ethical and Legal Considerations
Integrating ethical and legal considerations is another non-negotiable aspect of outcome-centric governance. GenAI initiatives must adhere to relevant regulations and ethical standards, including data privacy laws, intellectual property rights, and industry-specific regulations. Compliance with these standards is essential for mitigating legal risks and building trust with customers and stakeholders.
Promoting the ethical use of GenAI involves establishing principles prioritizing fairness, transparency, and accountability. Enterprises must proactively address potential biases in AI models, ensure alignment with organizational values, and maintain transparency in AI decision-making processes. By upholding ethical standards, enterprises can build trust, reduce reputational risks, and contribute to long-term sustainability and social responsibility.
Overcoming Challenges with Outcome-Centric Governance
Adopting an outcome-centric approach to governance is crucial for GenAI initiatives to deliver tangible results, but it often involves overcoming various obstacles. But these can be effectively managed with the right strategies and mindset.
Tech Mahindra and Microsoft AI experts help create value props, define roles, and prioritize use cases to solve customers' business problems. The team has defined principles and transparent governance structures that build trust in AI amongst all stakeholders.
Managing Uncertainty
The uncertainty around GenAI necessitates flexible governance frameworks for businesses that can adapt to emerging risks and opportunities. Enterprises must stay abreast of the latest advancements in AI technology and regulatory changes, to foster a culture of learning and adaptability. Continuous governance policy and practice updates empower enterprises to adeptly navigate uncertainty, fostering sustained success.
Balancing Control and Innovation
Striking a delicate balance between control and innovation is a key challenge in outcome-centric governance. While stringent control measures are essential for risk management
s, fostering innovation requires flexibility and experimentation. Enterprises must implement guided flexibility to address this challenge, setting clear boundaries for experimentation and exploration.One effective approach is to define 'safe zones' for innovation, where GenAI initiatives can be tested with minimal risk to critical operations. For example, an enterprise could designate a particular business unit or project to pilot new AI-driven solutions before scaling them organization-wide. By confining innovation within controlled environments, enterprises can minimize potential negative impacts while fostering a culture of experimentation and creativity.
Establishing rigorous testing and validation mechanisms is crucial for GenAI initiatives. Before deployment, techniques such as A/B testing, simulation environments, and sandboxing can help evaluate performance and robustness. Comprehensive testing protocols identify and mitigate potential risks early, enhancing the reliability and trustworthiness of the solutions.
Ensuring Scalability
Scalability poses another major challenge in outcome-centric governance, mainly as the use of GenAI grows within enterprises. As GenAI initiatives scale across departments, business units, and geographies, governance processes must evolve to handle increasing volumes of data, complexity, and operational demands.
To address these scalability challenges, enterprises can implement centralized governance supported by scalable infrastructure and tools. Centralized governance enables enterprises to establish consistent policies, procedures, and oversight mechanisms across diverse GenAI initiatives, facilitating efficient management and compliance. Additionally, deploying scalable infrastructure, such as cloud-based platforms and distributed computing resources, enables enterprises to accommodate growing computational demands and data volumes without compromising performance or reliability.
Furthermore, investing in automation and orchestration technologies can streamline governance processes and reduce administrative overhead. Automating routine tasks such as data ingestion, model training, and compliance monitoring improves efficiency, agility, and scalability while minimizing the risk of human error for enterprises.
Specific Metrics and Key Performance Indicators (KPIs)
To effectively monitor and evaluate the governance of GenAI initiatives, enterprises must establish clear, specific metrics and KPIs spanning various dimensions, including cost, performance, accuracy, compliance, and ethical considerations.
Cost Metrics
- Total Cost of Ownership (TCO): Includes hardware, software, maintenance, and operational costs associated with GenAI initiatives.
- Cost per Transaction/Request: Measures the cost efficiency of AI operations, enabling enterprises to optimize resource allocation and maximize ROI.
- Return on Investment (ROI): Compares the benefits gained from AI implementations to their associated costs, providing insights into the overall economic impact of GenAI initiatives.
Performance Metrics
- Latency: Measures the time AI systems take to respond to requests or process data, influencing AI-powered applications' responsiveness and user experience.
- Throughput: Quantifies the number of transactions or requests processed by AI systems within a given timeframe, indicating their capacity and scalability.
- System Uptime: Represents the percentage of time that AI systems are operational and available, reflecting their reliability and availability to end-users.
Accuracy Metrics
- Precision and Recall: Key metrics for evaluating the performance of AI models in specific tasks such as classification and prediction, providing insights into their effectiveness and reliability.
- F1 Score: The harmonic mean of precision and recall. It measures AI model performance across multiple dimensions.
- Error Rates: Including metrics such as false positives and false negatives, highlighting the accuracy and robustness of AI predictions and decisions.
Compliance Metrics
- Regulatory Compliance Rate: Measures the percentage of AI operations and outcomes that comply with relevant regulatory standards and legal requirements, ensuring adherence to industry regulations and guidelines.
- Audit Findings: Quantifies the number and severity of issues identified during compliance audits and reviews, indicating the effectiveness of governance controls and processes.
- Data Privacy Breaches: Tracks the number of incidents involving unauthorized access, disclosure, or misuse of sensitive data, assessing the effectiveness of data protection measures and privacy controls.
Ethical Considerations
- Bias and Fairness: Evaluate AI models and algorithms for biases and disparities in their outputs and decisions, ensuring fairness and equity across diverse user populations.
- Transparency and Explainability: Measures the degree to which AI systems can transparently and coherently explain their decisions, facilitating user trust and understanding.
- User Feedback: Solicits and analyzes user feedback regarding AI systems' ethical behavior and implications, enabling enterprises to address concerns and improve ethical standards.
Methodology for Continuous Evaluation and Improvement
Effective governance requires a structured method for continuous evaluation and improvement, encompassing several vital steps:
- Establish Baseline Metrics: Set baseline metrics and benchmarks for all KPIs before implementing GenAI initiatives, providing a reference point for future comparison and assessment.
- Regular Monitoring: Implement automated monitoring tools and dashboards to continuously track KPIs and performance metrics in real-time, enabling proactive identification of deviations and issues.
- Periodic Audits: Conduct regular audits and reviews to assess compliance with governance policies, regulatory standards, and ethical guidelines, identifying areas for improvement and corrective action.
- Feedback Loops: Establish feedback loops where human supervisors and stakeholders review AI outputs and decisions, providing insights and corrections to improve accuracy, fairness, and reliability.
- Iterative Improvements: Based on insights from monitoring, audits, and feedback, make iterative improvements to AI models, governance processes, and infrastructure, ensuring continuous enhancement and optimization.
- Training and Development: Invest in training and development programs to educate staff on best practices in GenAI governance, compliance, and ethical use, empowering them to address challenges and leverage opportunities effectively.
The Indispensable Role of Outcome-Centric Governance
Outcome-centric governance represents a fundamental paradigm shift in the governance of GenAI initiatives, that emphasizes strategic alignment, measurable outcomes, and stakeholder engagement. As GenAI continues to reshape the business landscape, enterprises must recognize the critical importance of outcome-centric governance in ensuring the success and sustainability of their AI initiatives. By prioritizing strategic alignment, flexibility, and ethical considerations, enterprises can navigate challenges, mitigate risks, and harness GenAI's transformative power to drive long-term growth and prosperity.
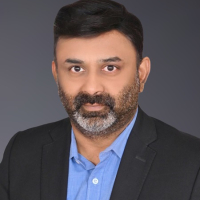
Rohit is a seasoned professional with over 26 years of experience in the IT industry, spanning various sectors. For the past decade, he has been at the forefront of innovation, utilizing AI technologies to address complex business challenges for clients across diverse domains and geographies. His expertise lies in defining strategies and spearheading AI-led transformations for clients.More
Rohit is a seasoned professional with over 26 years of experience in the IT industry, spanning various sectors. For the past decade, he has been at the forefront of innovation, utilizing AI technologies to address complex business challenges for clients across diverse domains and geographies. His expertise lies in defining strategies and spearheading AI-led transformations for clients. He firmly believes that purposeful AI can significantly transform business processes and uncover a wide range of opportunities to generate substantial business value. Currently, Rohit is working as Practice Head/Group Practice Head for the AI business unit at Tech Mahindra.
Less