Leveraging GenAI for Improving Resiliency & Predictability in Auto Finance
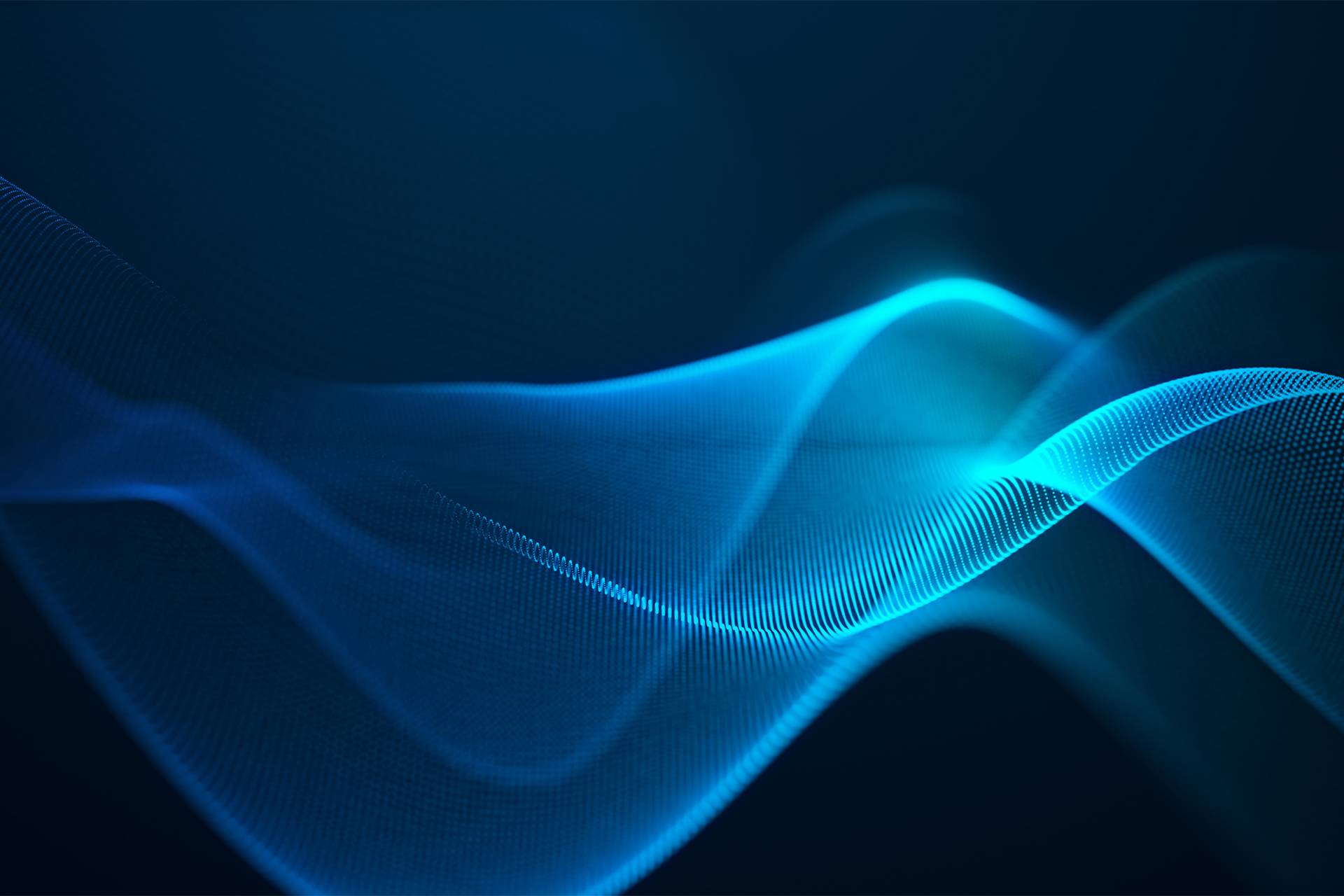
Generative AI (GenAI) is transforming the auto finance industry in several key areas, providing innovative solutions to enhance customer experience, streamline operations, and manage risks. It can generate synthetic data, automate processes, and provide valuable insights for decision-making. It helps revolutionize the auto finance industry by driving greater accuracy and speed, reducing the workload of financial professionals, and achieving better outcomes with improved efficiency.
According to a report by MarketResearch.biz, the global market size for GenAI in financial services is projected to reach approximately $9,475.2 million by 2032, marking a significant growth from $847.2 million in 2022. The market is expected to experience a compound annual growth rate (CAGR) of 28.1% during the forecast period from 2023 to 2032 (LeewayHertz, n.d.)1.
Current Challenges of the Auto Finance Industry
- Rising Interest Rates: This is a challenge for both consumers and lenders. Consumers will have to pay a higher interest while lenders try to maintain a competitive price.
- Delinquency and Default Risks: Economic conditions fluctuate, especially with inflation. The risk of loan delinquencies and default rises, leading to higher losses for lenders.
- Fraud and Cybersecurity: With the rise of digital auto finance platforms, fraud detection and prevention are becoming highly complicated. Cybersecurity risks and financial data breaches are increasing in finance and banking.
- Shifting Consumer Preferences: Modern consumers are moving towards alternative vehicle ownership models like leasing ride-sharing.
- EV Financing: Financing for EVs is becoming a distinct challenge due to higher upfront costs, uncertain resale values, and tech dynamics, which can complicate loan terms and depreciation predictions.
- Residual Value Risk: Lenders face difficulties in predicting the residual values of cars at the end of leases or loan terms, especially with rapid changes in vehicle technology and shifts in consumer demand for certain types of vehicles (EVs versus internal combustion engines).
- Supply Chain Disruptions: Global supply chain issues, such as semiconductor shortages, have affected vehicle production, reducing the inventory available for financing and increasing vehicle prices. This has made loans more expensive and increased the risk for both lenders and borrowers.
Factors Driving GenAI Usage in Auto Finance
Advanced Algorithms: Deep learning and reinforcement learning algorithms allow models to be trained on massive datasets, generating highly accurate predictions.
Increased Data Volume: With digitization underway at many finance captives, legacy silos are being dismantled, leading to data availability and standardization. Employing Generative AI techniques on newly available and abundant data provides new insights and predictions, which provide valuable information to guide decision-making in the finance industry.
Cost Reduction: GenAI facilitates automation, permitting streamlined operations and better resource allocation, resulting in significant cost savings for financial institutions. Across the banking industry, GenAI could deliver value equal to an additional $200 billion to $340 billion annually if the use cases are fully implemented (McKinsey & Company, 2024)2.
Business Challenges in GenAI Adoption
- Data Privacy and Compliance: Auto finance involves handling sensitive customer data. It is necessary to ensure that data privacy regulations (like GDPR or CCPA) are met while utilizing this data is a major concern. There is also the risk of synthetic data generation inadvertently exposing or recreating personal information.
- Integration with existing systems: Auto finance companies often depend on legacy systems that may not be compatible with advanced AI technologies. Integrating Generative AI into these systems can be technically challenging and expensive, requiring efficient IT resources and expertise.
- Model transparency and explainability: In auto finance, where regulatory scrutiny is high, there is a need for transparency and explainability in how AI models arrive at decisions, especially when it comes to risk assessment and fraud detection.
- Cost of Implementation: Developing and deploying Generative AI solutions can cost high to a company. This includes the cost of acquiring or developing the technology, training the staffs and maintaining the AI systems.
- Skill gaps: Implementing Generative AI requires specialized skills in data science, machine learning, and AI. There may be a shortage of qualified employees within the organization, making it necessary either to extensively train the existing employee or hiring new staff with the required expertise.
- Ethical and bias concerns: In auto finance, where decisions can affect people's financial futures, ensuring that AI models are fair and unbiased is very important. Addressing ethical concerns and avoiding discrimination in AI-driven decisions is a significant challenge.
By managing the adoption challenges efficiently, a company can use GenAI and provide multiple use cases to the market to solve current issues or enhance existing tasks. Let us look at a few GenAI use cases related to auto finance.
Use Cases of GenAI across the Auto Finance Value Chain
Origination
- Verification of Customer documentation
- Initial issues / concerns identification from received documents
Assessment & Underwriting
- Identification of document (Bank statement) tampering
- Tally debits and credits from bank statements to determine loan repayment eligibility
- System recommendation for go/ no go for loan approval
Contracting
- Contract simplification and summarization for customers
- Contract data migration to advanced CLM system from legacy system (IT use case)
Servicing
- Summarization of the cases and customer conversations for agent understanding
- Agent training by using GenAI
- Chatbot for customer service
Collections
- Delinquency models to predict customers at risk of missing repayment
- Key insights about customer & contract to Collections teams for nuanced collections strategy
Remarketing
- Verification if the engine numbers and VIN match in all documentations
- Vehicle description content creation for auction portals
Roadmap for GenAI Adoption
Adopting GenAI becomes crucial for staying competitive as the captive finance industry evolves. Auto finance companies can follow a few steps to prepare to integrate this technology into their operations.
Here are some key strategies to consider:
- Research Case Studies to Prepare and Strategize:
Research about successful case studies of GenAI implementation in the finance industry. Analyze how other organizations have leveraged this technology to gain insights and improve efficiency. Using these insights, develop a strategic plan for integrating a GenAI tool into your operations. - Find and Train Professionals for GenAI Adoption:
Building a team of professionals with good knowledge of GenAI technology is crucial. Invest in training programs and workshops to upskill the existing workforce and hire new talent. - Work Closely with IT Teams:
Collaboration between functional teams (collections, accounting, operations, risk management, legal, and compliance) and IT departments is integral for successful AI adoption. IT teams can provide the necessary infrastructure, data management systems, and security protocols to support implementation. Regular communication and coordination among these teams will ensure a smooth integration process. - Build or Buy a GenAI Tool:
Many organizations face this challenge: their data is a mess.
To overcome this obstacle, executives across industries must decide whether to build or buy their GenAI tool. Building large language models (LLMs) takes significant time, resources, and capital to effectively acquire, clean, and curate extensive data sets. For model training, one would need computational resources (i.e., hardware, software, cloud services), ramping personnel, maintenance, updates, etc. It’s better to buy a GenAI tool from an established service provider. - Pilot Programs:
Before fully implementing advancements across operations, it’s better to run pilot programs to test their effectiveness and identify any potential challenges. Initially, you may start with small-scale projects and gradually expand as you gain confidence in the technology. Monitor results closely and make necessary adjustments to optimize performance.
Conclusion
Auto finance companies and financial institutions are uniquely positioned to take an early lead in adopting GenAI. However, a company can quickly run its operations detrimentally without properly implementing guardrails and understanding the limitations and potential consequences of using GenAI. By aligning GenAI with strategic objectives, a company can position itself ahead of the curve, ready to capitalize on GenAI's true commercial potential.
Industry leaders must pay attention to pinpointing specific areas where GenAI can genuinely provide commercial value to their businesses in the present setup by:
- Fostering a continuous learning and adaptation culture to stay current on advancements in GenAI and industry trends.
- Promote an environment encouraging experimentation, risk-taking, and creative problem-solving to substitute innovation.
- Prioritize transparency and accountability in GenAI decision-making processes to build trust among stakeholders.
- To foster trust and understanding, communicate openly about GenAI's use, including its limitations, biases, and ethical considerations.
- Implement robust governance frameworks and mechanisms for auditing GenAI systems to ensure accountability and compliance with regulatory requirements.
- Develop ethical guidelines and principles for developing, deploying, and using GenAI systems.
- Provide training and support to help employees effectively adapt to GenAI systems.
- Encourage employee feedback and inputs on GenAI-related initiatives, empowering them to contribute insights and expertise about development and improvement.
Ramashree S has overall 9 years of experience in IT Industry. She holds a bachelor’s degree in engineering from AMC Engineering College Bangalore, affiliated to Visvesvaraya Technological University (VTU), Belagavi.