The Engine Behind AI: AI Accelerator
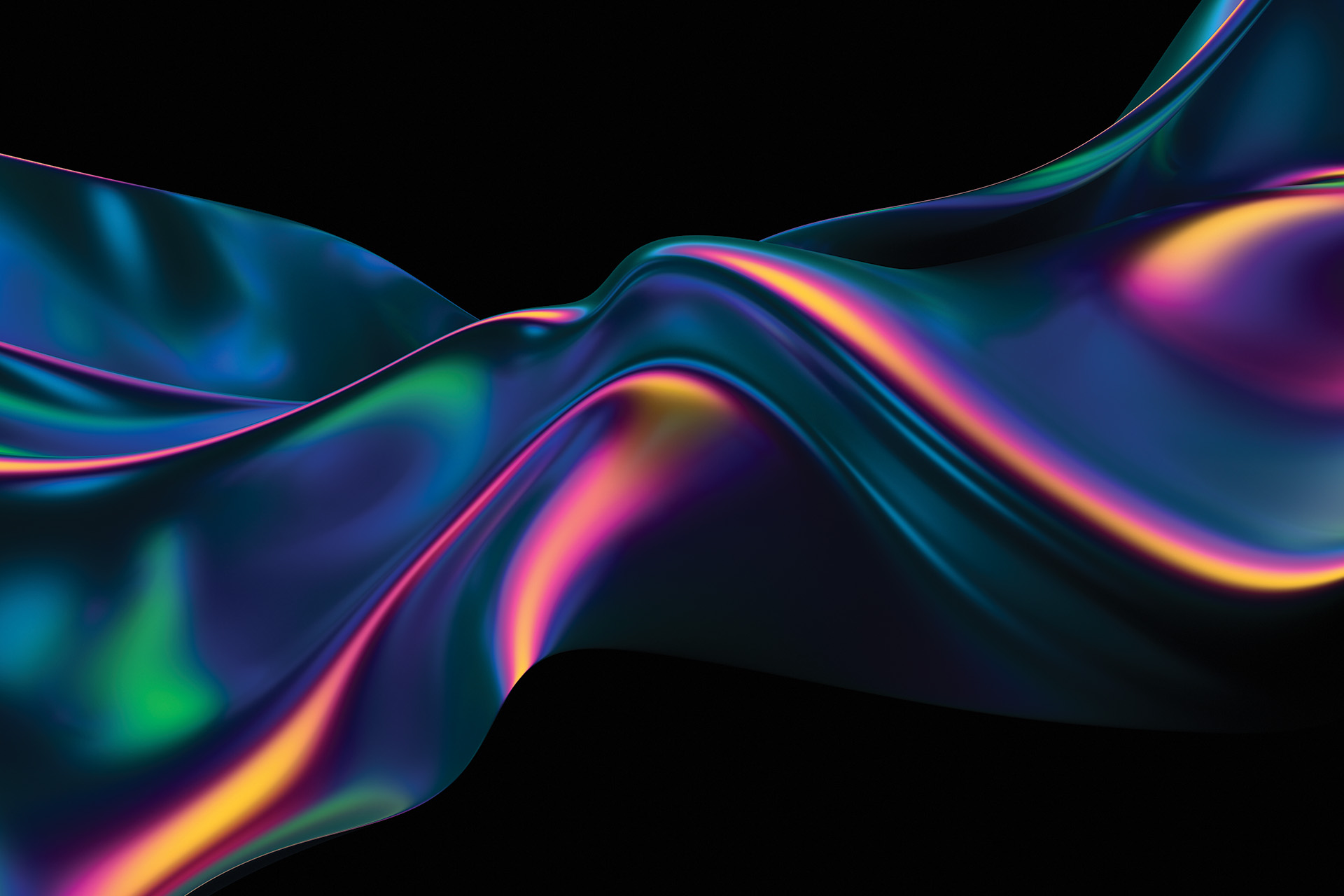
The last few years have witnessed a profound shift in operational models across global industries. The reason? The exponential evolution of AI. Foundational models of AI in 2024 encompass various capabilities, including, but not limited to, computer code, images, video, and audio. As a direct impact, we can see a plethora of AI-assisted innovations in healthcare, automotive, finance, and more. A McKinsey study (2023) reveals that the new generations of AI can inject $2.6 – $4.4 trillion across all industries combined1.
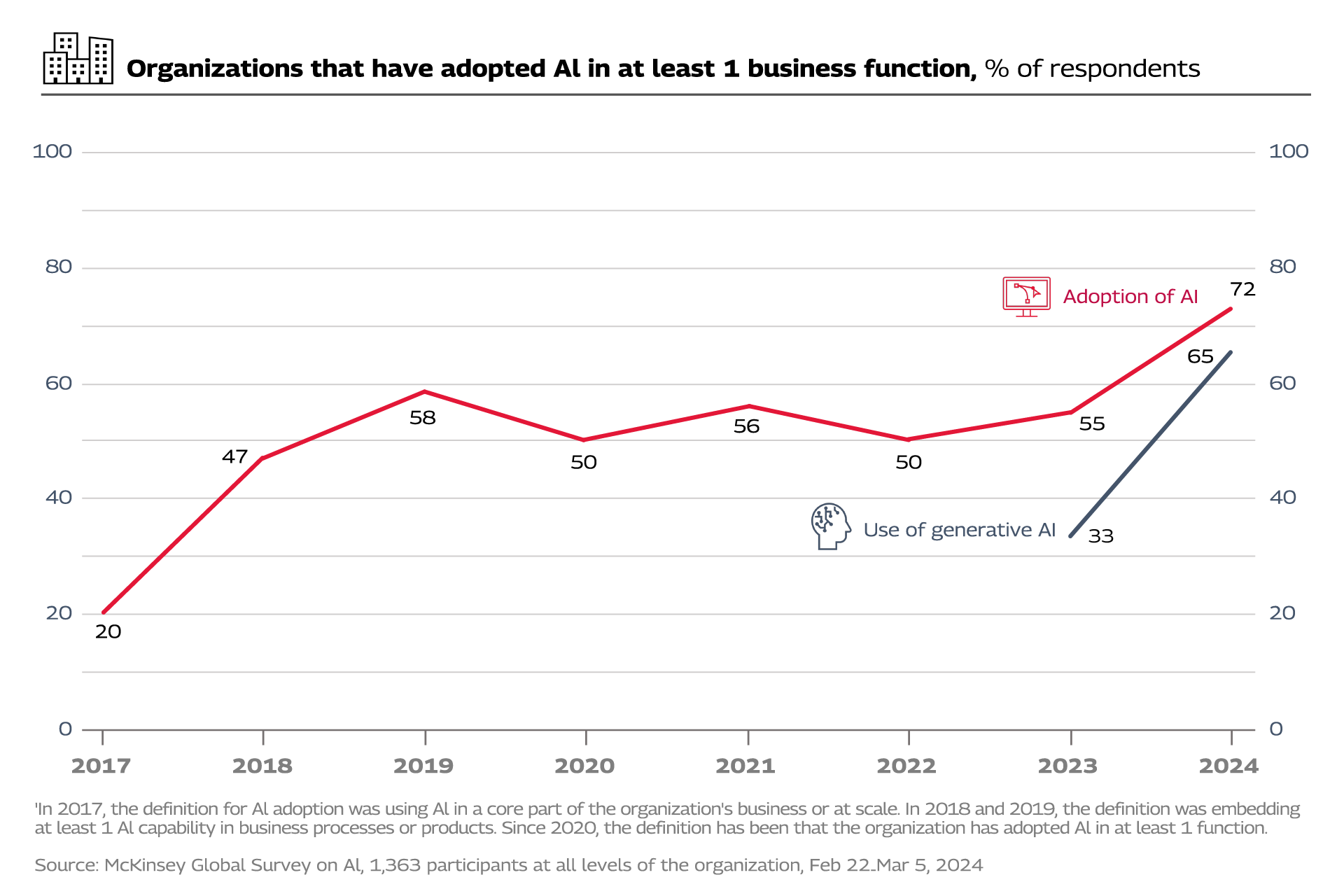
|
Source: The state of AI in early 2024
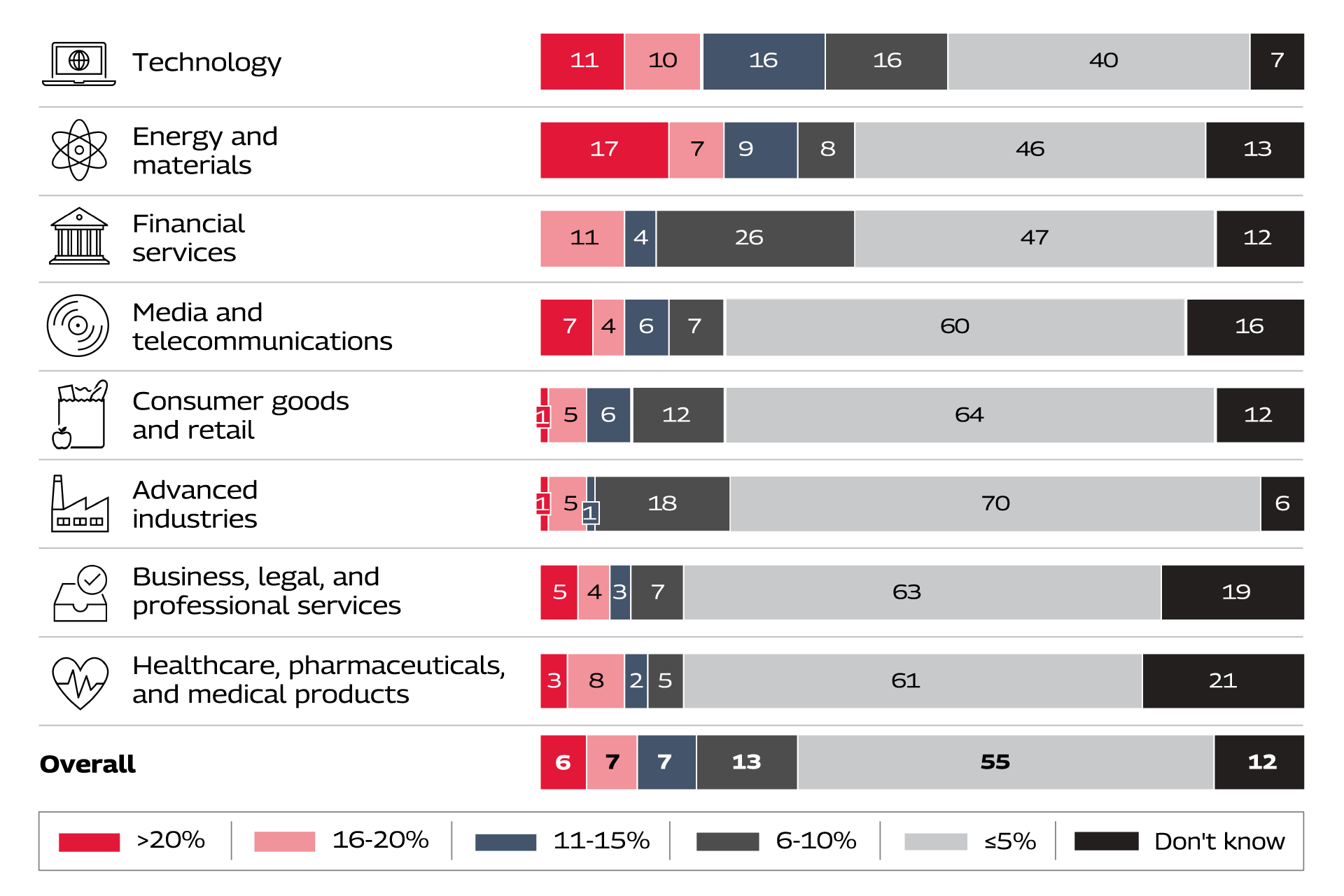
|
Source: The state of AI in early 2024
Specialized hardware components – artificial intelligence (AI) accelerators – are catalyzing this timely AI revolution. Conventional CPUs are not optimized for complex operations like matrix multiplications and parallel processing, especially in machine learning and deep learning. In contrast, AI accelerators deliver faster processing, improved energy efficiency, and better scalability.
Reasons You Should Use AI Accelerators
- Speed
- Efficiency
- Scalability
Key Use Cases for AI Accelerators
- Training and Inference in Machine Learning: AI accelerators are crucial for the training and inference of models like deep neural networks (DNNs) and convolutional neural networks (CNNs). These models require large datasets and enormous computational power, which GPUs, TPUs, and other accelerators provide.
- Edge AI: Edge AI refers to performing AI tasks on devices at the network's edge—closer to the data source rather than in centralized cloud servers. Accelerators like NPUs in smartphones and IoT devices enable real-time AI inference, reducing latency and improving efficiency.
- Natural Language Processing (NLP): Accelerators speed up the training and inference of large language models (LLMs) like GPT and BERT, allowing businesses and researchers to train models faster and deploy real-time NLP systems.
- Autonomous Vehicles: AI accelerators are pivotal in developing autonomous vehicles, which rely on real-time sensor data processing, such as LIDAR and cameras, to make split-second driving decisions.
Different Types of AI Accelerators
However, all AI accelerators are not built the same. Certain accelerators function well in some use cases while others demand a different accelerator. To unlock the most from AI accelerators, and subsequently the AI tools, we need to understand different accelerators and their functions. Let’s break it down:
- Graphics Processing Units (GPUs): Today, the most commonly used AI accelerator is the GPU. It was primarily designed for rendering images in computer graphics but was found to be useful for AI as well due to its capacity for handling massive parallel computations. It is an effective accelerator for training deep learning models that require running a huge number of operations in parallel for large datasets. As a result, GPUs can be used for both research and commercial AI applications, such as training neural networks for image recognition or natural language processing. The most notable GPUs in the present market are NVIDIA's CUDA-enabled GPUs (A100/B200 and DGX Cloud), Intel Gaudi 3, and AMD's Radeon GPUs among others.
- Tensor Processing Units (TPUs): Google has purpose-built TPUs for growing AI workloads of modern-day operations. Therefore, they offer an opportunity to optimize those tasks involved in deep learning. TPUs also specialize well in those models of TensorFlow, open source from Google. They help users speed up large-scale training and inference as intended.
- Application-Specific Integrated Circuits (ASICs): ASICs are custom-built application-specific chips. This means even if they might perform poorly than general-purpose processors in certain areas, ASICs can outperform them when it comes to the specific task they are designed for. In this regard, Google's TPU, which is optimized for AI and deep learning tasks, can be considered a form of ASIC.
- Field-Programmable Gate Arrays (FPGAs): FPGAs present an adaptable and easily programmable path to AI acceleration. Unlike rigid processors such as ASICs, FPGAs can be reprogrammed for various tasks, making them ideal for applications requiring flexibility. Some common FPGAs currently available are Xilinx FPGAs and Intel's Altera FPGAs.
- Neural Processing Units (NPUs): NPUs are specialized processor cores for neural network computing. NPUs are, in general, integrated directly into mobile devices or edge-computing systems and can provide AI inference on the local device, not in a cloud. Applications that call for low latency and efficiency, such as real-time AI in autonomous systems, are perfect use cases for NPUs. At present, Apple's Neural Engine, Qualcomm's Hexagon DSP, and Huawei's NPU in Kirin chipsets are a few of the leading NPUs in use.
Leading AI Accelerator Technologies
In addition to this broad classification of AI accelerators, there are many other types available in the current market and they fit into many unique use cases. Here’s a breakdown of all the popular AI accelerators available in the market:
- Cerebras CS-3
The Cerebras CS-3 is a next-generation AI accelerator that is 57x more powerful than the strongest GPUs available today. It offers a groundbreaking computing power of 125 petaflops with 900,000 AI-optimized cores. As a result, it can handle massive models with up to 24 trillion parameters, making it ideal for training large-scale models like GPT-5 and beyond. - Graphcore IPU (Intelligence Processing Unit)
The Graphcore IPU is designed to handle fine-grained parallelism, offering significant compute density with 1,216 processing cores per chip. Its unique architecture efficiently handles sparse neural networks, making it ideal for reinforcement learning and sparse data applications.
The Future of AI Accelerators: What Lies Ahead?
AI accelerators are still evolving, and their future promises even more powerful capabilities. Here are a few trends and innovations expected in the coming years:
- Quantum AI Accelerators
Quantum computing is the next hardware frontier in AI. Currently still in its infancy, quantum computers promise to bring forth the next stage of the AI revolution by solving problems that even today’s best accelerators cannot handle. Accelerators for quantum AI could enormously shorten the time it takes to train huge AI models and open doors to brand-new applications. - Accelerators in Consumer Electronics
Even more consumer devices will undoubtedly feature AI accelerators in the coming months. Whether a wearable device that is equipped with real-time health diagnostics or AR glasses analyzing surroundings in real-time, AI-based consumer electronics will change how we use technology in our lives. This trend dictates more AI accelerators in consumer electronics.
In addition to this, energy-efficient AI models are also gaining popularity. The high demand for the AI accelerator will lead to a substantial focus on the development of an energy-efficient model. These models can save operational costs while reducing the negative carbon footprint issues related to massive AI deployment. The major hardware companies will further improvise in developing architectures related to more power efficiency such as the use of neuromorphic computing and neural structures mimicking the human brain along with AI accelerators. So only one question remains. Are you ready for the future?
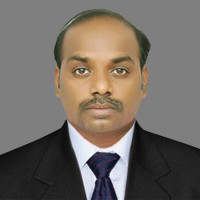
Babukannan is a distinguished technology professional with a Bachelor's degree in Electronics Instrumentation and a Master's degree in Applied Electronics. Currently pursuing a Ph.D. in Computer Vision, he brings over 23 years of extensive experience in the IT industry.
MoreBabukannan is a distinguished technology professional with a Bachelor's degree in Electronics Instrumentation and a Master's degree in Applied Electronics. Currently pursuing a Ph.D. in Computer Vision, he brings over 23 years of extensive experience in the IT industry.
His expertise encompasses a wide range of technologies, including embedded systems, AI and AI accelerators, IoT and sensors, multimedia (audio and video) engineering, video streaming, and conferencing. Babukannan has successfully contributed to various sectors, including semiconductors, geospatial, mobile communications, enterprise solutions, and entertainment. He also has a strong knowledge of parallel computing and multicore architecture.
Babukannan has demonstrated a commitment to driving innovation and operational efficiency throughout his career, making him a key leader in the domain.
Less