Barriers Towards Enterprise AI Adoption - The Infrastructure Trinity
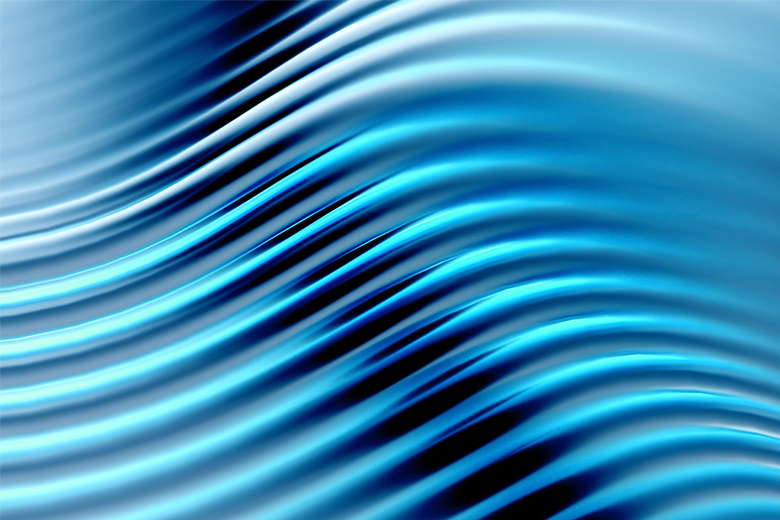
Point of View Series - Part 4
We started this blog series with a topic on right use cases followed by data privacy, quality, and quantity. In this blog post, we would be focusing on the three foundational enterprise infrastructural elements, namely – compute, storage, and networking
Barrier # 4 – The Infrastructure Trinity
The trinity of compute, storage, and networking need each other to survive and thrive.
Realizing the AI dream requires fast, parallel, and complex processing engines which computing processors like graphics processing units (GPUs) help to build. These engines need high volume, variety and velocity of structured and unstructured data requiring an appropriate storage solution as a curated repository, ready to be summoned quickly. Finally, great compute and storage solutions will be of no value without the power of networking conduits. These are the enterprise sensory pathways of routers, cables and switches which form the core for high bandwidth and low latency communication between the processing units and various enterprise end points enabling real time sensing and fast decision making.
Each of these three infrastructural elements are a pillar of strength of their own but, together, they form a formidable foundation for something far greater. However, if misaligned or insufficient, they can also significantly stifle an organization’s AI ambitions.
Let’s understand these barriers better.
- The Compute Conundrum: One of the primary barriers to AI adoption is the sheer computational power required to train and deploy complex AI models. Traditional computing architectures often struggle to keep up with the insatiable appetite of AI algorithms for processing power which also drives up associated energy consumptions. This mismatch can lead to prolonged training times, suboptimal model performance, high costs and ultimately, a reluctance to invest in AI initiatives.
- The Storage Strain: The exponential growth of data, a crucial fuel for AI, has put immense pressure on storage infrastructure. Companies often find themselves grappling with the challenge of managing and storing vast amounts of structured and unstructured data required for AI model training and inference. Inadequate storage capacity, slow data retrieval, and inefficient data management can significantly hinder the progress of AI projects.
- The Networking Nemesis: Effective AI deployment relies heavily on seamless data flow and communication between various components of the AI ecosystem. However, outdated, or insufficient networking infrastructure can create bottlenecks, leading to latency, data loss, and suboptimal performance of AI applications. This challenge is particularly acute in scenarios where AI models need to be deployed at the edge, where low-latency and high-bandwidth connectivity are essential.
Well, where there are problems, we also find solutions.
Multiple technological advancements in these fields are making it easier to design solutions to address these challenges.
The compute side evolution is being driven by advanced and, at times, purpose built specialized hardware like GPUs, tensor processing units (TPUs) and field programmable gate arrays (FPGAs) which are optimized for specific AI workloads thereby offering necessary computational power to process large data sets and complex algorithms better and faster than traditional processors. Choosing hybrid and multi cloud environments to optimize cost and performance by distributing AI workloads and experimenting with high performance computing (HPC), edge computing and quantum computing capabilities are also helping to address opportunities and challenges associated with this pillar. While the GPUs are available from a technological perspective, but the demand vs supply is still a challenge. With quantum computing becoming reality, the compute barriers will be addressed.
On the storage side we are seeing high performance storage solutions like all flash arrays and non-volatile memory express (NVMe) come in to offer low latency and high throughput capabilities. Implementing a multi-tiered storage solution from high-performance flash storage for hot data to cost-effective object storage for cold data, is allowing enterprises to balance performance and cost. Storage optimization technologies such as deduplication, compression, and thin provisioning are being used to significantly reduce storage requirements and costs. Cloud storage solutions are a great solution to go storage as-a-service and pay only for what being used and not for carrying idle, future capacities. Storage solutions are also becoming more nuanced with multi modal storage options handling various data types including images, videos, text, and machine sensor data
Finally, the network side advancements are also quite encouraging. Advances in CPU-GPU communications and GPU-GPU communications, fiber optic technology and the deployment of 5G networks to provide High bandwidth connections are stepping up support for large scale data analytics, video processing and machine learning needs. Software defined networking (SDN) developments can now better allow networks to be more flexible and adaptable while providing dynamic management of varying AI workloads. Edge networking solutions are also coming in to support IoT and autonomous vehicle kind of edge computing use cases bringing data processing closer to source of data generation, minimizing data travel needs and reducing latency. Other technologies like network function virtualization (NFV) and advanced network analytics (ANA) are replacing traditional specialized hardware-based network functions with software running on standard hardware while bringing in improved network management and operations control. Such smarter networks can predict and respond to changes in demand, identify and mitigate security threats and optimize network performance in real time.
To sum up, the evolution of technologies to address infrastructure related barriers is marked by the development of specialized hardware like GPUs, TPUs, and FPGAs for efficient processing of AI tasks, and the adoption of hybrid and multi-cloud environments to distribute AI workloads. High-performance storage solutions like all-flash arrays and NVMe are being employed for better speed and capacity, with multi-tiered storage systems optimizing costs. Advances in networking, including NVLink, fiber optics, 5G, SDN, and edge networking, support large-scale AI operations, while technologies like NFV and ANA improve network flexibility and real-time management. These solutions are not only helping to address the challenges faced by the trinity solutions individually but are also helping to address the integrated impacts on availability, scalability, sustainability, and reliability.
Our next few blogs will be focusing on exploring the transformative potential of AI models, platforms, and tools. We will cover how they pave the way for seamless AI integration, overcoming traditional barriers to adoption and unlocking new horizons in business efficiency and growth.
If you missed the first post of the series, you could find it here
Know More
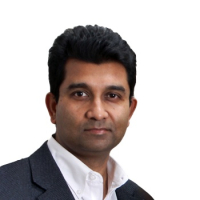
Partha currently manages an industry business group of strategic lighthouse customer relationships within the TME business unit at Tech Mahindra. He brings over two and a half decades of experience in discrete manufacturing and technology consulting services covering North America, Europe, and Asia Pacific markets across automotive, consumer electronics, semiconductor, networking, ISVs, gaming and financial services domains.More
Partha currently manages an industry business group of strategic lighthouse customer relationships within the TME business unit at Tech Mahindra. He brings over two and a half decades of experience in discrete manufacturing and technology consulting services covering North America, Europe, and Asia Pacific markets across automotive, consumer electronics, semiconductor, networking, ISVs, gaming and financial services domains. In his professional career he has helped to design and execute multiple business value impact strategies while managing strategic client relationships and industry vertical focused P&L Management responsibilities.
Less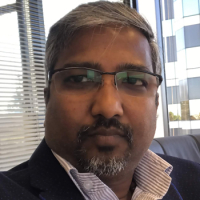
Dr. Pandian Angaiyan heads Tech Mahindra’s technology business as the chief technology officer (CTO) and is based out of San Jose office. He has three decades of experience in incubating and leading computing businesses based on niche technologies, which gives him the right tools to lead disruptive digital transformation initiatives for Tech Mahindra’s customers.More
Dr. Pandian Angaiyan heads Tech Mahindra’s technology business as the chief technology officer (CTO) and is based out of San Jose office. He has three decades of experience in incubating and leading computing businesses based on niche technologies, which gives him the right tools to lead disruptive digital transformation initiatives for Tech Mahindra’s customers. In his previous role, he has lead the cloud Innovation business for a global consulting company where he has played the role of cloud transformation partner for several customers, helping define their cloud strategy, building minimum viable products, and eventually transforming them into full- fledged solutions. Dr. Pandian has two decades of experience in various computing technologies starting from embedded systems all the way to hyper scale architectures.
Dr. Pandian has a Ph.D., in symbolic and numeric computational algorithms for real time applications from the Indian Institute of Science, Bangalore and has a masters of technology in computer engineering from Mysore University, India.
Less