Barriers towards Enterprise AI Adoption - AI Trust and Safety
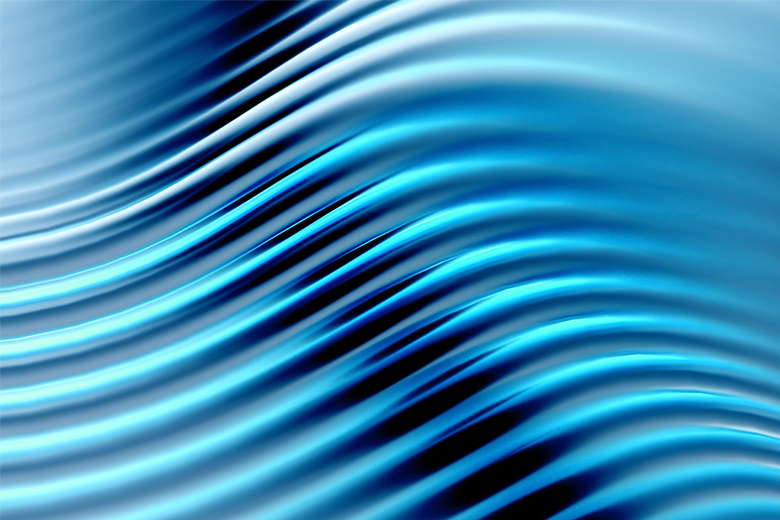
Point of View Series - Part 6
In our “Barriers towards Enterprise AI Adoption” blog series, we have so far covered the “functional” and “technical” challenges of developing AI adoption strategies. In our previous installment, we delved into AI models, frameworks, tools, and platforms—the technical backbone of AI systems. You can check out the previous installment in this series here.
Now, we will begin exploring “operational” barriers, starting with Trust and Safety.
Barrier # 6 - AI Trust and Safety
AI (Artificial Intelligence) in today’s world is transforming enterprise strategy by driving efficiency, enhancing decision-making, and developing innovative business models. However, as AI systems become integral to critical decision-making across product design, business operations, and customer engagements, “Trust and Safety” becomes a key barrier to adoption too.
Understanding the Barriers and Their Significance
“Trust” in AI means stakeholders’ confidence—from employees to customers, and regulators—in the technology’s ability to operate reliably, ethically, legally, and transparently free from bias or manipulation. “Safety”, on the other hand, implies that AI systems function securely without vulnerabilities, comply with regulations, protect data privacy, and do not cause any damage to the business or stakeholders.
Flawed AI models can cause financial losses, harm individuals, and lead to regulatory violations. A lack of trust in the AI systems results in ineffective use by employees and reluctance to adopt from customers. Biased decisions, privacy violations, or manipulation by malicious actors can damage trust, reputation, and brand identity.
The path to implementing appropriate measures is riddled with challenges as follows:
- Transparency and Explainability: Many AI models, especially deep learning systems, operate as “black boxes,” which makes their decision-making process opaque. This lack of transparency and explainability is a significant barrier to trust, especially in regulated sectors like finance and healthcare.
- Algorithmic Bias and Fairness: AI systems can inadvertently reinforce biases when trained on data that contains inherent biases, as well as through biases introduced during the training process itself. This leads to unfair decisions in critical areas like hiring, credit scoring, and law enforcement. Biases can occur more often, especially in the reinforced learning models, due to how the reward parameters are set up.
- Data Privacy and Security: AI systems require vast data, often sensitive or personal. Ensuring secure data handling and compliance with regulations like GDPR and CCPA is a critical challenge. The lack of data traceability in the entire training and inference process provides barriers to realizing the full potential of AI systems.
- Ethical Uncertainty: Advanced AI systems raise ethical questions, especially in surveillance, military applications, and automated decision-making. Ensuring AI aligns with ethical standards and organizational values is an ongoing challenge.
- Malicious Actor Attacks: AI systems can be manipulated by adversarial attacks, posing severe risks in areas like autonomous vehicles, cybersecurity, and fraud detection. The lack of mature protection mechanisms against malicious actor attacks slows down the adoption of AI systems.
The regulatory landscape for AI is still evolving, and enterprises often find themselves navigating a complex web of guidelines, standards, and laws varying by jurisdiction. This uncertainty can make it difficult for organizations to develop AI strategies that are compliant across all markets. However, these regulations can also help enterprises stay safe and overcome concerns in the areas mentioned above.
- EU AI Act: Categorizes AI systems by risk levels, with stringent requirements for high-risk applications in critical areas like infrastructure and employment.
- GDPR: Ensures transparency and accountability, mandating clear explanations for AI-driven decisions that impact individuals' rights and privacy.
- US Initiatives: Include sector-specific guidelines and frameworks like the AI Bill of Rights and NIST’s AI Risk Management Framework (AI RMF) to ensure responsible AI development and use.
- ISO Standards: Emphasize ethical, trustworthy, and safe AI implementation, setting international benchmarks for AI governance.
- National AI Strategies: Countries like China and the UK are developing strategies balancing innovation with ethical and regulatory frameworks.
While businesses face numerous challenges and risks in adopting AI technology amidst stringent regulatory compliance demands, many enterprises are beginning to realize the significant value AI can bring. They are navigating these complexities to unlock its tremendous promise and potential.
Let’s briefly touch upon a few of them:
- Explainable AI Models: Some companies have started investing in tools to make AI decision-making more understandable, explainable, and auditable.
- AI Auditing Services: Some organizations offer regular fairness checks to mitigate bias, ensuring AI models remain equitable over time.
- Strengthening AI Security: Investments in advanced cybersecurity tools protect against adversarial attacks, using techniques like differential privacy and federated learning.
- Engagement with Regulators: Firms collaborate with regulatory bodies to shape AI standards and stay ahead of the evolving compliance requirements.
- Developing Ethical AI Guidelines: Inspired by frameworks like the EU’s Ethics Guidelines, organizations are creating their own standards to ensure responsible AI development and deployment.
While multiple paths of evolution are influenced by the availability of tools, capabilities, and regulations, we have attempted to make a few predictions and recommendations about future trends based on the actions and initiatives we see in the industry today.
- Regulations: As AI integrates into business operations, regulations will continue to evolve. Global harmonization efforts are expected to simplify cross-border compliance.
- Governance: Organizations will need robust governance frameworks, including ethical guidelines, fairness checks, transparency protocols, and safety audits, to build stakeholder trust and comply with regulations on an ongoing basis.
- Humanized Tech: The future will see AI designed around human needs, safety, and ethical considerations, incorporating insights from ethics, sociology, and cognitive science.
- Explainability: Research into interpretable and explainable models and analytics will enhance transparency and trust in AI systems.
- Technologies: Quantum computing, edge AI, and federated learning will offer new ways to improve trust and safety. Investments in AI-specific cybersecurity will protect against threats.
- Ethics: Establishing dedicated AI ethics boards will provide ongoing guidance on ethical AI development.
- Sustainability: Research and development into energy-efficient training and inference with alternative compute engines and architectures will reduce carbon emissions and operational costs, fostering broader adoption.
Trust and safety are critical challenges in AI adoption, but they are not insurmountable. By proactively addressing these concerns, enterprises can build trust, mitigate risks, and unlock AI’s full potential sustainably. Robust governance, explainable AI technologies, and staying updated with regulatory changes will help organizations navigate these challenges effectively.
The future of AI lies in balancing innovation with responsible deployment, ensuring that AI systems are powerful, trustworthy, and safe. As regulations and technologies evolve, organizations prioritizing trust and safety will be better positioned for sustainable growth and innovation.
As we keep venturing into other critical operational areas in our future posts, you will see a more holistic picture about “Enterprise barriers towards AI adoption” emerge. Keep reading and sharing your thoughts!
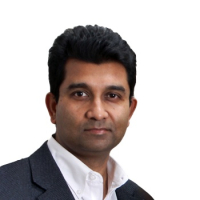
Partha currently manages an industry business group of strategic lighthouse customer relationships within the TME business unit at Tech Mahindra. He brings over two and a half decades of experience in discrete manufacturing and technology consulting services covering North America, Europe, and Asia Pacific markets across automotive, consumer electronics, semiconductor, networking, ISVs, gaming and financial services domains.More
Partha currently manages an industry business group of strategic lighthouse customer relationships within the TME business unit at Tech Mahindra. He brings over two and a half decades of experience in discrete manufacturing and technology consulting services covering North America, Europe, and Asia Pacific markets across automotive, consumer electronics, semiconductor, networking, ISVs, gaming and financial services domains. In his professional career he has helped to design and execute multiple business value impact strategies while managing strategic client relationships and industry vertical focused P&L Management responsibilities.
Less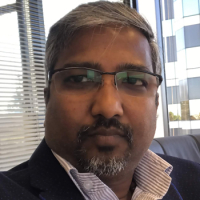
Dr. Pandian Angaiyan heads Tech Mahindra’s technology business as the chief technology officer (CTO) and is based out of San Jose office. He has three decades of experience in incubating and leading computing businesses based on niche technologies, which gives him the right tools to lead disruptive digital transformation initiatives for Tech Mahindra’s customers.More
Dr. Pandian Angaiyan heads Tech Mahindra’s technology business as the chief technology officer (CTO) and is based out of San Jose office. He has three decades of experience in incubating and leading computing businesses based on niche technologies, which gives him the right tools to lead disruptive digital transformation initiatives for Tech Mahindra’s customers. In his previous role, he has lead the cloud Innovation business for a global consulting company where he has played the role of cloud transformation partner for several customers, helping define their cloud strategy, building minimum viable products, and eventually transforming them into full- fledged solutions. Dr. Pandian has two decades of experience in various computing technologies starting from embedded systems all the way to hyper scale architectures.
Dr. Pandian has a Ph.D., in symbolic and numeric computational algorithms for real time applications from the Indian Institute of Science, Bangalore and has a masters of technology in computer engineering from Mysore University, India.
Less