Barriers Towards Enterprise AI Adoption - AI Models, Framework, Tools, and Platforms
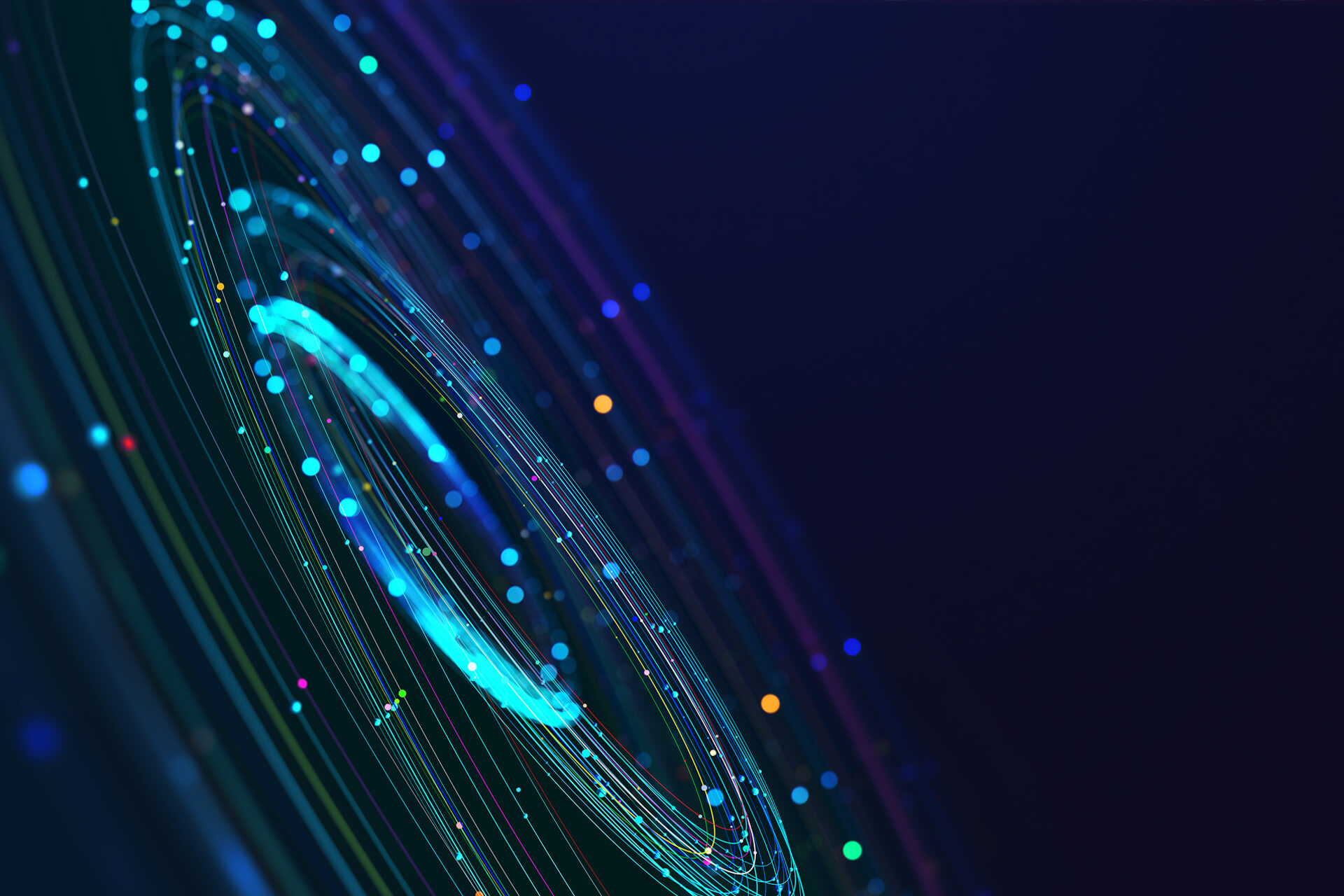
Point of View Series - Part 5
In our previous blog posts, we discussed the various elements, such as use cases, data, and infrastructure, that are required to be understood and managed well for enterprises to succeed in their AI journey. These are crucial foundation elements that must be addressed first before we touch on the core technical elements in this post – the AI models, framework, tools, and platforms.
Enterprises face significant barriers in adopting AI due to the complexity of selecting and developing suitable models, frameworks, tools, and platforms that align with their business needs. Factors such as problem complexity, data quality, solution scalability and compatibility, resource efficiency, costs, ethical and legal implications add to the difficulty while potentially becoming barriers to success. This post delves deeper into these elements and provides recommendations for due diligence to help mitigate such selection barriers.
Let’s start with the definitions, first.
Barrier # 5 – AI Models, Framework, Tools, and Platforms
AI models are algorithms with learning parameters trained on curated data to perform specific tasks such as classification, regression, object detection, language understanding, summarization, generation, and more. They provide the core capability for making predictions and generating insights and can be specialized and fine-tuned for specific tasks, processes, and industries. These algorithms are machine learning models (supervised or unsupervised), deep learning models or Reinforcement Learning models. There are multiple options for choosing the right models. From the availability and readiness point of view there are models 1) which are pre-trained and publicly available, 2) pre-trained models but deployed privately, 3) pre-trained open-source models, 4) un-trained open-source models, 5) partially trained open-source models and 6) custom models.
Depending on the use case and business case, the appropriate model needs to be chosen. Once a particular model is chosen, there are multiple parameters within a particular model, which needs to be chosen to get the right outcome.
AI framework and tools are software products and frameworks that facilitate various stages of AI lifecycle like data collection, pre-processing, model selection, model training, evaluation, deployment, and monitoring. They enhance productivity and bring in efficiency through automation while also extending the integration with existing workflows and systems. There are multiple frameworks and tools available in the market. Choosing the right framework and tools depends on the hardware infrastructure used for both training and inference, selection of models, context in which the models are being trained and deployed for interference, and more. While choosing the right model is an involved task, choosing the right framework and tools also needs closer attention from enterprise’s roadmap perspective.
AI platforms are integrated environments that provide a comprehensive suite of tools, services and sometimes even models for the end-to-end lifecycle of AI development – from data ingestion to model deployment and monitoring. They provide collaboration, agility, scalability, and reliability for enterprise grade AI implementations while reducing the need for multiple disparate tools with the aim to offer seamless end to end solutions. These platforms provide functional capabilities in the entire lifecycle of the models and data as well as operational capabilities such as security, governance, observability, monitoring, performance, and more. While the platforms makes it easy from rolling out the AI capabilities within an enterprise, but still choosing the right platform needs decision making as many platforms are available and these platforms are at various levels of maturity as well as differing capabilities.
So, these three elements complement each other with platforms offering the environment, tools enabling the lifecycle processes and both working to make the models focus on the tasks assigned to them to create value.
Now let’s talk about the assessment factors which are key. Enterprises must consider those before selecting the ones “best suited” to meet their needs.
Broadly there are ten such factors which require due diligence – whether the selection involves a model, framework, tool, or a platform. These factors / needs are, in no specific order , the following – (1) data quality and availability to work with (2) feature and functionality fit including integration needs with existing systems (3) talent and skill gaps to manage them (4) performance and scalability expectations (5) customization and flexibility needed (6) total cost of ownership and ROI (7) security, privacy and compliance desired including bias and fairness (8) vendor and community support for maintenance and regular updates (9) user experience, adoption, and change management (10) alignment with business objectives and business roadmap.
To overcome these challenges, enterprises should invest in data management and governance, foster AI talent development, prioritize scalability and integration from the outset, and proactively engage with policymakers and ethical AI frameworks. Additionally, partnering with experienced AI service providers can help enterprises navigate these complexities more effectively.
Conclusion
With this post, we have completed addressing the functional and technical appreciation elements to be managed by enterprises during their AI journeys. In the upcoming posts, we would like to address other important elements like trust and safety, regulations and compliance, talent and learning, organizational change management and financial assessment and ROI.
If you missed the first post of the series, you could find it here
Know More
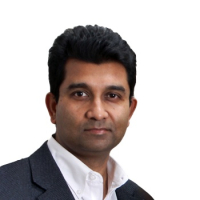
Partha currently manages an industry business group of strategic lighthouse customer relationships within the TME business unit at Tech Mahindra. He brings over two and a half decades of experience in discrete manufacturing and technology consulting services covering North America, Europe, and Asia Pacific markets across automotive, consumer electronics, semiconductor, networking, ISVs, gaming and financial services domains.More
Partha currently manages an industry business group of strategic lighthouse customer relationships within the TME business unit at Tech Mahindra. He brings over two and a half decades of experience in discrete manufacturing and technology consulting services covering North America, Europe, and Asia Pacific markets across automotive, consumer electronics, semiconductor, networking, ISVs, gaming and financial services domains. In his professional career he has helped to design and execute multiple business value impact strategies while managing strategic client relationships and industry vertical focused P&L Management responsibilities.
Less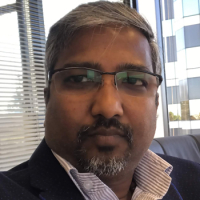
Dr. Pandian Angaiyan heads Tech Mahindra’s technology business as the chief technology officer (CTO) and is based out of San Jose office. He has three decades of experience in incubating and leading computing businesses based on niche technologies, which gives him the right tools to lead disruptive digital transformation initiatives for Tech Mahindra’s customers.More
Dr. Pandian Angaiyan heads Tech Mahindra’s technology business as the chief technology officer (CTO) and is based out of San Jose office. He has three decades of experience in incubating and leading computing businesses based on niche technologies, which gives him the right tools to lead disruptive digital transformation initiatives for Tech Mahindra’s customers. In his previous role, he has lead the cloud Innovation business for a global consulting company where he has played the role of cloud transformation partner for several customers, helping define their cloud strategy, building minimum viable products, and eventually transforming them into full- fledged solutions. Dr. Pandian has two decades of experience in various computing technologies starting from embedded systems all the way to hyper scale architectures.
Dr. Pandian has a Ph.D., in symbolic and numeric computational algorithms for real time applications from the Indian Institute of Science, Bangalore and has a masters of technology in computer engineering from Mysore University, India.
Less