Moving Past the GenAI Honeymoon Phase: Key Factors to Consider for Scaling Adoption
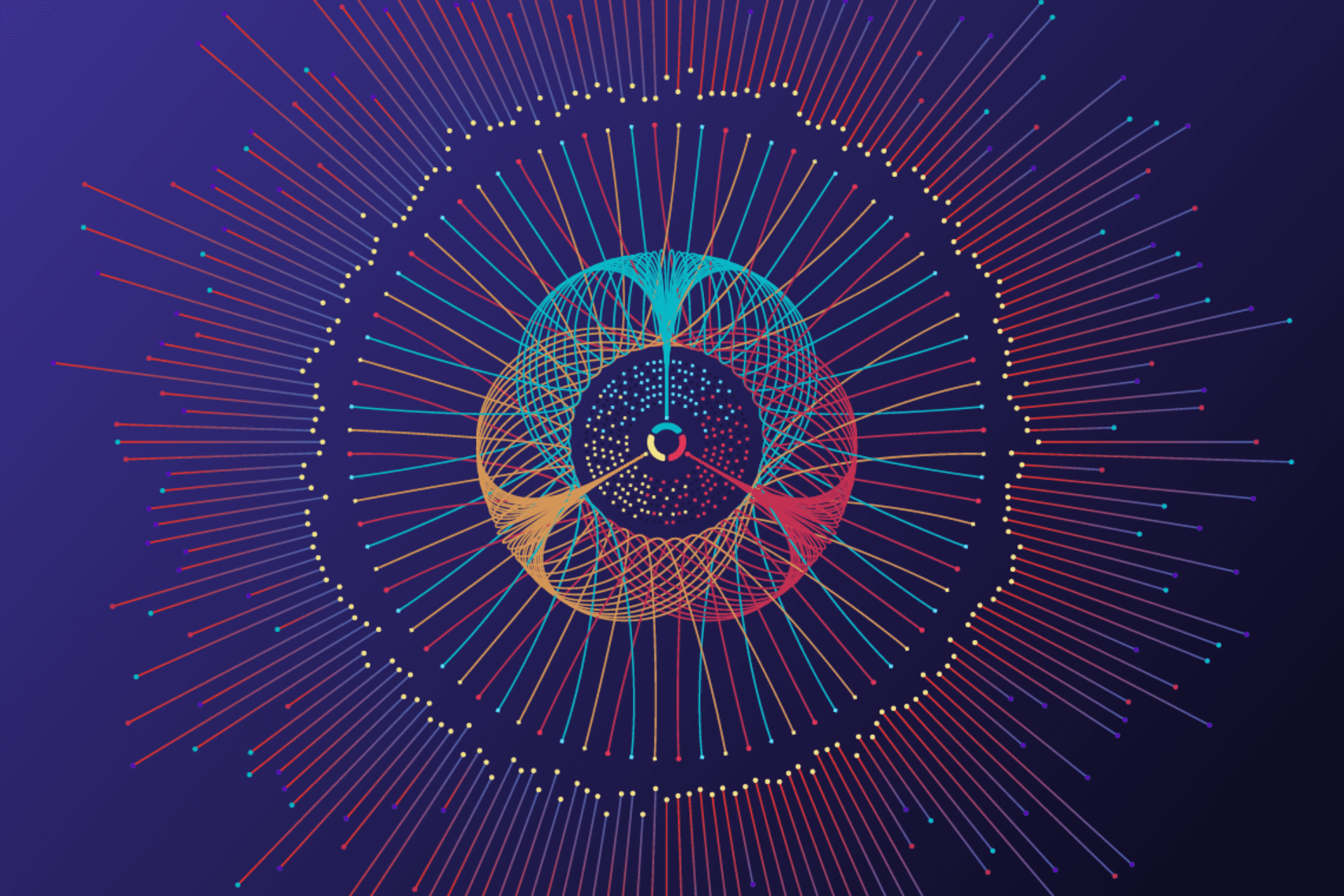
Generative AI (GenAI) has captured the imagination of industries across the globe. Its ability to create content, solve complex problems through self-learning algorithms, and present endless possible use cases has brought excitement among businesses and consumers alike. However, as organizations move beyond the initial "honeymoon phase" of experimentation and early adoption, scaling GenAI in a way that delivers sustained value requires thoughtful planning, strategy, robust implementation framework, and determination. This is precisely why only 11% of the companies have adopted GenAI at scale.
To fully unlock the potential of GenAI at scale, enterprises need to consider several key factors that go beyond early experimentation by establishing foundations for scalable technology and rethinking operational workflows. From managing expectations to addressing data governance, security, and workforce readiness, let’s explore the core considerations for organizations aiming to elevate their GenAI efforts.
Defining an AI Strategy Aligned with Business Objectives
Artificial Intelligence (AI) has the potential to be a powerful tool for increasing productivity, enhancing efficiency, and driving innovation provided it aligns with the organization's business objectives. Today, many GenAI applications and use cases are fragmented, often addressing isolated problems with minimal impact if implemented in silos. Therefore, it becomes essential for CXOs to define a comprehensive AI roadmap with the following considerations in mind:
- Short-term and long-term organizational goals
- An AI strategy with tangible benefits, milestones, or KPIs
- Following the SMART ideology - Specific, Measurable, Attainable, Relevant, and Time-bound
Only this level of planned innovation can lead to measurable outcomes, such as reduced costs, improved productivity, and increased customer satisfaction or operational efficiency.
Monitoring, Analyzing, and Iterating
Given the cost and time involved in developing and implementing AI into day-to-day operations, organizations must continually monitor, analyze, and iterate to ensure a positive return on investment (ROI). CXOs need to monitor the collection of accurate, high-quality, and diverse datasets since they influence the overall accuracy and reliability of the GenAI models. The data needs to be analyzed using the correct algorithms to generate the necessary outcomes and make adjustments required to improve efficiency and accuracy.
Operationalizing AI with Scalable Tools and Platforms
AI is no longer native to new-age companies, organizations across different sectors are operationalizing AI to achieve business objectives. For enterprises across sectors to improve and maximize business values using AI, it is necessary to operationalize it at scale. However, it is easier said than done, as deploying a few AI models is far simpler than running an entire organization powered by AI.
Organizations that are committed to AI adoption need to adopt Machine Learning Operations (MLOps), a newer discipline through which we can establish the necessary ecosystem to facilitate the rapid, safe, and efficient development of GenAI models. MLOps also ensures that the AI models are continuously monitored, updated, and maintained using data pipelines, performance tracking, and lifecycle management, leveraging scalable AI platforms provided by various cloud service providers (CSPs).
Building a Strong Data Infrastructure and Governance Framework
Data is the fuel that powers all AI systems. Reliable and diverse data improves the learning process, helping AI models to understand the various tasks at hand. As organizations try to scale AI adoption, they are impacted either by the diversity of the available data set or the lack of the necessary infrastructure to store, develop, and implement the models. This is because ensuring data accessibility and security is crucial for GenAI models to perform efficiently.
While scaling the GenAI adoption enterprises need to ensure the necessary governance framework, policies, and controls are in place to ensure the quality, integrity, and security of the data throughout its lifecycle. Governance protocols should be set up to protect sensitive information, enforce ethical usage, and meet compliance requirements.
Ethical Considerations and Responsible AI
While implementing GenAI is crucial for business success, organizations must consider safety to build trust among users, since GenAI models rely on data collected from its users to improve accuracy. Organizations must clarify data ownership, storage, and usage of the data. They also should enforce transparent policies regarding data sharing, storage, and utilization to foster trustworthiness, ensure ethical consideration, and promote responsible use of AI.
Cross-functional Collaboration and Workforce Transformation
Given the complexity of scaling GenAI models, it needs collaboration among multiple teams and departments within an organization to prevent AI initiatives from being developed in silos and to encourage constant feedback from all stakeholders. Leaders need to define the AI strategy and technical teams need to clearly explain the capabilities and limitations of AI across the organization for easier implementation and adaptation.
Organizations need to invest in developing and upskilling resources, enriching them with the latest developments in the field of AI to avoid major roadblocks in adaptation. Organizations must ensure that employees clearly understand the application of GenAI in automating routine processes. Further, AI adoption should be demonstrated and democratized across all functions within the organization to ensure adaptability.
Conclusion
As organizations transition from the initial enthusiasm surrounding GenAI to long-term implementation, organizations need to focus on building sustainable GenAI practices that drive meaningful impact. Successful scaling of GenAI models is not about just deploying these models at scale but also ensuring that these developments align with the overall business objectives. Ultimately, a well-defined strategy will enhance operational efficiency, and support innovation and growth in an increasingly data-driven world.
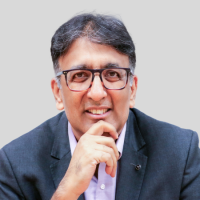
With over 28 years of experience in IT and Technology Industry, including leadership roles in various organizations like Infosys, Wipro, Aricent, and GlobalLogic Rohit Madhok is a distinguished, high-impact business and technology leader at Tech Mahindra with a diverse skill set that encompasses managing and executing large & complex digital transformation projects.More
With over 28 years of experience in IT and Technology Industry, including leadership roles in various organizations like Infosys, Wipro, Aricent, and GlobalLogic Rohit Madhok is a distinguished, high-impact business and technology leader at Tech Mahindra with a diverse skill set that encompasses managing and executing large & complex digital transformation projects. Rohit is a well-respected media spokesperson with active engagements in key industry forums such as the Mobile World Congress, TM Forum, Qualcomm DX Summit, NASSCOM Events, ARM Summit, and others. He has been widely and exclusively featured as an established thought leader in Times Job, The Industry Outlook, Dataquest, TechGig, Mobile Magazine UK, TechCircle, NCN Magazine, EFY Magazine, LinkedIn Live, and many more.
Less